NVIDIA’s wide range of AI frameworks, unlocked by NVIDIA AI Enterprise, accelerate the development and deployment of AI projects. Leveraging pre-configured frameworks removes many of the manual tasks and complexity associated with software development, enabling you to deploy your AI models faster as each framework is tried, tested and optimised for NVIDIA GPUs. The less time spent developing, the greater the ROI on your AI hardware and data science investments.
Scan’s unique AI ecosystem provides all you need to deploy your applications, from initial proof of concept, data science expertise, specialist hardware and professional services.
Start your AI journey by exploring the range of AI frameworks below.
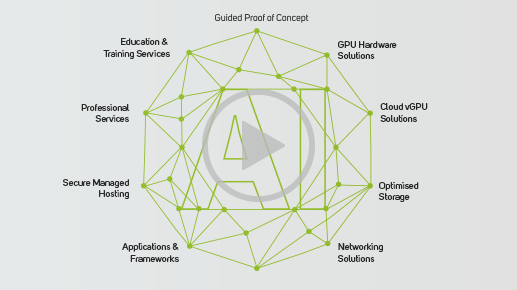
AI Frameworks
Explore the range of NVIDIA frameworks, sorted alphabetically by use case, that can help speed up developing your AI applications.
What is NVIDIA DRIVE?
Use the NVIDIA DRIVE open-source framework for developing and deploying autonomous vehicles. The NVIDIA DRIVE AGX Developer Kit makes up the in-car solution that for hardware, software, and applications, whilst NVIDIA DRIVE Infrastructure encompasses the complete datacentre solution needed to develop autonomous driving technology—from raw data collection and neural network development to testing and validation in simulation.
Is NVIDIA DRIVE Right for You?
To ensure NVIDIA DRIVE is the right application for your datasets and challenges, Scan AI offers a free guided proof of concept (POC) trial. Our cloud-based GPU-accelerated hardware is located in UK datacentres and can be accessed securely from anywhere.
You are able to use your own data and will be supported by our expert data scientists to ensure you get the most from trying NVIDIA DRIVE.
Leverage our Expertise
After your POC, our in-house team of data scientists and developers can identify and extract value from your data, speeding up application deployment.
Our team will work to provide analytical insight from your structured and unstructured data, defining a clear strategy which will be presented to your organisation, identifying quick wins, potential risks and include suggestions on how to minimise bottlenecks. Consultancy of this type is often pivotal in shaping your hardware plans too, as data driven projects always offer clearer system requirements when choosing AI infrastructure optimised for NVIDIA DRIVE.
Additionally, we will train your teams to expand data science knowledge within your organisation.
Further your Knowledge
Scan AI is a certified NVIDIA Deep Learning Institute (DLI) provider. We offer a wide range of instructor-led courses to help your organisation get the most from GPU-accelerated hardware.
NVIDIA DRIVE physics-based models will scale rapidly, so the ‘Data Parallelism: How to Train Deep Learning Models on Multiple GPUs’ course is ideal to expand your understanding and prepare for your projects.
Get ready for NVIDIA DRIVE
An NVIDIA AI Enterprise subscription is required to make the most of NVIDIA’s AI frameworks. Instead of assembling thousands of co-dependent libraries and APIs, AI Enterprise provides a full software stack and up to 24x7 technical support. AI Enterprise is licensed per GPU and is available on all AI systems from Scan.
Running NVIDIA DRIVE software will require different hardware configurations for the development and training phases. Our health-check consultancy services verifies the suitability of your existing infrastructure to effectively run NVIDIA DRIVE, with our experts offering advice on what upgrades to make if required.
AI Infrastructure for NVIDIA DRIVE
The NVIDIA DRIVE AGX Orin Developer Kit provides hardware, software and sample applications needed for the development of production-level autonomous vehicle applications. It is powered by the DRIVE OS SDK, a reference operating system and associated software stack that includes DriveWorks.
Custom-built AI servers from Scan 3XS Systems and NVIDIA DGX appliances, combined with PEAK:AIO software defined storage servers are the perfect way to train with NVIDIA DRIVE. These systems can be configured with up to eight NVIDIA GPUs and are fine-tuned by our hardware engineers for performance, reliability and low-noise. The custom software stack includes NVIDIA DRIVE, operating system and management tools.
NVIDIA DRIVE projects can also be developed, trained and scaled in a virtual environment using the Scan AI & Compute Cloud. This on-demand service has been developed from the ground up for intensive GPU-accelerated workloads and offers bespoke cloud instances to suit your individual projects, that can flex as your requirements develop and evolve.
Get the most from your AI Infrastructure
Ensuring your NVIDIA DRIVE infrastructure remains at its optimum is key to your applications continued evolution and expansion. The Scan AI Ecosystem includes a range of professional services including installation, configuration and monitoring alongside consulting for cyber security and end-to-end project management.
If necessary, we can arrange hosting of your AI infrastructure with a range of datacentre partners, based either in the UK or in a variety of Scandinavian countries to take advantage of renewable energy sources. We also provide colocation and relocation services to best suit your business and data compliance needs.
The nature of energy production in the UK often results in surplus renewable power being stranded in between the wind farms and the grid. This energy can be utilised by modular datacentres located where the surplus energy is generated, unlocking low-cost compute power and reduced costs compared to building a new permanent datacentre.
Share your NVIDIA DRIVE Success
Partnering with us for a case study increases brand exposure through coordinated joint-marketing with Scan and NVIDIA.
VIEW OUR CASE STUDIES >Get Started with NVIDIA DRIVE
What is NVIDIA ACE?
Use the NVIDIA Avatar Cloud Engine (ACE) open-source framework to bring digital avatars to life with generative AI. The NVIDIA ACE suite of technologies helps developers build their own digital human solutions from the ground up, or use ACE in conjunction with NVIDIA Merlin to design interactive digital assistants for customer service, or NVIDIA Riva and Maxine develop digital avatars for real-time communication.
Is NVIDIA ACE Right for You?
To ensure NVIDIA ACE is the right application for your datasets and challenges, Scan AI offers a free guided proof of concept (POC) trial. Our cloud-based GPU-accelerated hardware is located in UK datacentres and can be accessed securely from anywhere.
You are able to use your own data and will be supported by our expert data scientists to ensure you get the most from trying NVIDIA ACE.
Leverage our Expertise
After your POC, our in-house team of data scientists and developers can identify and extract value from your data, speeding up application deployment.
Our team will work to provide analytical insight from your structured and unstructured data, defining a clear strategy which will be presented to your organisation, identifying quick wins, potential risks and include suggestions on how to minimise bottlenecks. Consultancy of this type is often pivotal in shaping your hardware plans too, as data driven projects always offer clearer system requirements when choosing AI infrastructure optimised for NVIDIA ACE.
Additionally, we will train your teams to expand data science knowledge within your organisation.
Further your Knowledge
Scan AI is a certified NVIDIA Deep Learning Institute (DLI) provider. We offer a wide range of instructor-led courses to help your organisation get the most from GPU-accelerated hardware.
NVIDIA ACE physics-based models will scale rapidly, so the ‘Data Parallelism: How to Train Deep Learning Models on Multiple GPUs’ course is ideal to expand your understanding and prepare for your projects.
Get ready for NVIDIA ACE
An NVIDIA AI Enterprise subscription is required to make the most of NVIDIA’s AI frameworks. Instead of assembling thousands of co-dependent libraries and APIs, AI Enterprise provides a full software stack and up to 24x7 technical support. AI Enterprise is licensed per GPU and is available on all AI systems from Scan.
Running NVIDIA ACE software will require different hardware configurations for the development and training phases. Our health-check consultancy services verifies the suitability of your existing infrastructure to effectively run NVIDIA ACE, with our experts offering advice on what upgrades to make if required.
AI Infrastructure for NVIDIA ACE
Custom-built AI workstations from Scan 3XS Systems are the perfect way to start developing with NVIDIA ACE. Workstations can be configured with up to six NVIDIA RTX GPUs and are fine-tuned by our hardware engineers for performance, reliability and low-noise. The custom software stack includes NVIDIA ACE, Ubuntu operating system and Docker for containerised workloads.
Custom-built AI servers from Scan 3XS Systems and NVIDIA DGX appliances, combined with PEAK:AIO software defined storage servers are the perfect way to train with NVIDIA ACE. These systems can be configured with up to eight NVIDIA GPUs and are fine-tuned by our hardware engineers for performance, reliability and low-noise. The custom software stack includes NVIDIA ACE, operating system and management tools.
NVIDIA ACE projects can also be developed, trained and scaled in a virtual environment using the Scan AI & Compute Cloud. This on-demand service has been developed from the ground up for intensive GPU-accelerated workloads and offers bespoke cloud instances to suit your individual projects, that can flex as your requirements develop and evolve.
Get the most from your AI Infrastructure
Ensuring your NVIDIA ACE infrastructure remains at its optimum is key to your applications continued evolution and expansion. The Scan AI Ecosystem includes a range of professional services including installation, configuration and monitoring alongside consulting for cyber security and end-to-end project management.
If necessary, we can arrange hosting of your AI infrastructure with a range of datacentre partners, based either in the UK or in a variety of Scandinavian countries to take advantage of renewable energy sources. We also provide colocation and relocation services to best suit your business and data compliance needs.
The nature of energy production in the UK often results in surplus renewable power being stranded in between the wind farms and the grid. This energy can be utilised by modular datacentres located where the surplus energy is generated, unlocking low-cost compute power and reduced costs compared to building a new permanent datacentre.
Share your NVIDIA ACE Success
Partnering with us for a case study increases brand exposure through coordinated joint-marketing with Scan and NVIDIA.
VIEW OUR CASE STUDIES >Get Started with NVIDIA ACE
What is NVIDIA Morpheus?
Use the NVIDIA Morpheus open-source framework to create applications for sorting, analysing and classifying large volumes of streaming cybersecurity data. Morpheus uses AI to reduce the time and cost associated with identifying, capturing and acting on threats, whilst also extending human analysts’ capabilities with generative AI by automating real-time analysis and responses, producing synthetic data to train AI models that identify risks accurately, and run varied ‘what-if’ scenarios.
Is NVIDIA Morpheus Right for You?
To ensure NVIDIA Morpheus is the right application for your datasets and challenges, Scan AI offers a free guided proof of concept (POC) trial. Our cloud-based GPU-accelerated hardware is located in UK datacentres and can be accessed securely from anywhere.
You are able to use your own data and will be supported by our expert data scientists to ensure you get the most from trying NVIDIA Morpheus.
Leverage our Expertise
After your POC, our in-house team of data scientists and developers can identify and extract value from your data, speeding up application deployment.
Our team will work to provide analytical insight from your structured and unstructured data, defining a clear strategy which will be presented to your organisation, identifying quick wins, potential risks and include suggestions on how to minimise bottlenecks. Consultancy of this type is often pivotal in shaping your hardware plans too, as data driven projects always offer clearer system requirements when choosing AI infrastructure optimised for NVIDIA Morpheus.
Additionally, we will train your teams to expand data science knowledge within your organisation.
Further your Knowledge
Scan AI is a certified NVIDIA Deep Learning Institute (DLI) provider. We offer a wide range of instructor-led courses to help your organisation get the most from GPU-accelerated hardware.
NVIDIA Morpheus physics-based models will scale rapidly, so the ‘Data Parallelism: How to Train Deep Learning Models on Multiple GPUs’ course is ideal to expand your understanding and prepare for your projects.
Get ready for NVIDIA Morpheus
An NVIDIA AI Enterprise subscription is required to make the most of NVIDIA’s AI frameworks. Instead of assembling thousands of co-dependent libraries and APIs, AI Enterprise provides a full software stack and up to 24x7 technical support. AI Enterprise is licensed per GPU and is available on all AI systems from Scan.
Running NVIDIA Morpheus software will require different hardware configurations for the development and training phases. Our health-check consultancy services verifies the suitability of your existing infrastructure to effectively run NVIDIA ACE, with our experts offering advice on what upgrades to make if required.
AI Infrastructure for NVIDIA Morpheus
Custom-built AI workstations from Scan 3XS Systems are the perfect way to start developing with NVIDIA Morpheus. Workstations can be configured with up to six NVIDIA RTX GPUs and are fine-tuned by our hardware engineers for performance, reliability and low-noise. The custom software stack includes NVIDIA Morpheus, Ubuntu operating system and Docker for containerised workloads.
Custom-built AI servers from Scan 3XS Systems and NVIDIA DGX appliances, combined with PEAK:AIO software defined storage servers are the perfect way to train with NVIDIA ACE. These systems can be configured with up to eight NVIDIA GPUs and are fine-tuned by our hardware engineers for performance, reliability and low-noise. The custom software stack includes NVIDIA Morpheus, operating system and management tools.
NVIDIA Morpheus projects can also be developed, trained and scaled in a virtual environment using the Scan AI & Compute Cloud. This on-demand service has been developed from the ground up for intensive GPU-accelerated workloads and offers bespoke cloud instances to suit your individual projects, that can flex as your requirements develop and evolve.
Get the most from your AI Infrastructure
Ensuring your NVIDIA Morpheus infrastructure remains at its optimum is key to your applications continued evolution and expansion. The Scan AI Ecosystem includes a range of professional services including installation, configuration and monitoring alongside consulting for cyber security and end-to-end project management.
If necessary, we can arrange hosting of your AI infrastructure with a range of datacentre partners, based either in the UK or in a variety of Scandinavian countries to take advantage of renewable energy sources. We also provide colocation and relocation services to best suit your business and data compliance needs.
The nature of energy production in the UK often results in surplus renewable power being stranded in between the wind farms and the grid. This energy can be utilised by modular datacentres located where the surplus energy is generated, unlocking low-cost compute power and reduced costs compared to building a new permanent datacentre.
Share your NVIDIA Morpheus Success
Partnering with us for a case study increases brand exposure through coordinated joint-marketing with Scan and NVIDIA.
VIEW OUR CASE STUDIES >Get Started with NVIDIA Morpheus
What is NVIDIA RAPIDS?
Use the NVIDIA RAPIDS open-source framework to increase data analytics performance by orders of magnitude at scale across data pipelines. Built on NVIDIA CUDA-X AI, RAPIDS combines years of experience development in graphics, machine learning, deep learning, high-performance computing (HPC), to ease the complexities of working with GPUs and the behind-the-scenes communication protocols within the datacentre architecture, enabling a simple way to accelerate data science workflows.
Is NVIDIA RAPIDS Right for You?
To ensure NVIDIA RAPIDS is the right application for your datasets and challenges, Scan AI offers a free guided proof of concept (POC) trial. Our cloud-based GPU-accelerated hardware is located in UK datacentres and can be accessed securely from anywhere.
You are able to use your own data and will be supported by our expert data scientists to ensure you get the most from trying NVIDIA RAPIDS.
Leverage our Expertise
After your POC, our in-house team of data scientists and developers can identify and extract value from your data, speeding up application deployment.
Our team will work to provide analytical insight from your structured and unstructured data, defining a clear strategy which will be presented to your organisation, identifying quick wins, potential risks and include suggestions on how to minimise bottlenecks. Consultancy of this type is often pivotal in shaping your hardware plans too, as data driven projects always offer clearer system requirements when choosing AI infrastructure optimised for NVIDIA RAPIDS.
Additionally, we will train your teams to expand data science knowledge within your organisation.
Further your Knowledge
Scan AI is a certified NVIDIA Deep Learning Institute (DLI) provider. We offer a wide range of instructor-led courses to help your organisation get the most from GPU-accelerated hardware.
NVIDIA RAPIDS physics-based models will scale rapidly, so the ‘Data Parallelism: How to Train Deep Learning Models on Multiple GPUs’ course is ideal to expand your understanding and prepare for your projects.
Get ready for NVIDIA RAPIDS
An NVIDIA AI Enterprise subscription is required to make the most of NVIDIA’s AI frameworks. Instead of assembling thousands of co-dependent libraries and APIs, AI Enterprise provides a full software stack and up to 24x7 technical support. AI Enterprise is licensed per GPU and is available on all AI systems from Scan.
Running NVIDIA RAPIDS software will require different hardware configurations for the development and training phases. Our health-check consultancy services verifies the suitability of your existing infrastructure to effectively run NVIDIA ACE, with our experts offering advice on what upgrades to make if required.
AI Infrastructure for NVIDIA RAPIDS
Custom-built AI workstations from Scan 3XS Systems are the perfect way to start developing with NVIDIA RAPIDS. Workstations can be configured with up to six NVIDIA RTX GPUs and are fine-tuned by our hardware engineers for performance, reliability and low-noise. The custom software stack includes NVIDIA RAPIDS, Ubuntu operating system and Docker for containerised workloads.
Custom-built AI servers from Scan 3XS Systems and NVIDIA DGX appliances, combined with PEAK:AIO software defined storage servers are the perfect way to train with NVIDIA RAPIDS. These systems can be configured with up to eight NVIDIA GPUs and are fine-tuned by our hardware engineers for performance, reliability and low-noise. The custom software stack includes NVIDIA Morpheus, operating system and management tools.
NVIDIA RAPIDS projects can also be developed, trained and scaled in a virtual environment using the Scan AI & Compute Cloud. This on-demand service has been developed from the ground up for intensive GPU-accelerated workloads and offers bespoke cloud instances to suit your individual projects, that can flex as your requirements develop and evolve.
Get the most from your AI Infrastructure
Ensuring your NVIDIA RAPIDS infrastructure remains at its optimum is key to your applications continued evolution and expansion. The Scan AI Ecosystem includes a range of professional services including installation, configuration and monitoring alongside consulting for cyber security and end-to-end project management.
If necessary, we can arrange hosting of your AI infrastructure with a range of datacentre partners, based either in the UK or in a variety of Scandinavian countries to take advantage of renewable energy sources. We also provide colocation and relocation services to best suit your business and data compliance needs.
The nature of energy production in the UK often results in surplus renewable power being stranded in between the wind farms and the grid. This energy can be utilised by modular datacentres located where the surplus energy is generated, unlocking low-cost compute power and reduced costs compared to building a new permanent datacentre.
Share your NVIDIA RAPIDS Success
Partnering with us for a case study increases brand exposure through coordinated joint-marketing with Scan and NVIDIA.
VIEW OUR CASE STUDIES >Get Started with NVIDIA RAPIDS
What is NVIDIA Modulus?
Use the NVIDIA Modulus open-source framework to build, train and fine-tune Physics-ML models using a simple Python interface. It empowers engineers to construct AI models that combine physics-driven causality with simulation and observed data, enabling real-time predictions. Modulus offers a variety of approaches for training models, from physics informed neural networks (PINNs) to data-driven architectures such as neural operators.
Modulus supports the creation of large-scale digital twin models across various physics domains, from computational fluid dynamics and structural mechanics to electromagnetics.
Is NVIDIA Modulus Right for You?
To ensure NVIDIA Modulus is the right application for your datasets and challenges, Scan AI offers a free guided proof of concept (POC) trial. Our cloud-based GPU-accelerated hardware is located in UK datacentres and can be accessed securely from anywhere.
You are able to use your own data and will be supported by our expert data scientists to ensure you get the most from trying NVIDIA Modulus.
Leverage our Expertise
After your POC, our in-house team of data scientists and developers can identify and extract value from your data, speeding up application deployment.
Our team will work to provide analytical insight from your structured and unstructured data, defining a clear strategy which will be presented to your organisation, identifying quick wins, potential risks and include suggestions on how to minimise bottlenecks. Consultancy of this type is often pivotal in shaping your hardware plans too, as data driven projects always offer clearer system requirements when choosing AI infrastructure optimised for NVIDIA Modulus.
Additionally, we will train your teams to expand data science knowledge within your organisation.
Further your Knowledge
Scan AI is a certified NVIDIA Deep Learning Institute (DLI) provider. We offer a wide range of instructor-led courses to help your organisation get the most from GPU-accelerated hardware.
NVIDIA Modulus physics-based models will scale rapidly, so the ‘Data Parallelism: How to Train Deep Learning Models on Multiple GPUs’ course is ideal to expand your understanding and prepare for your projects.
Get ready for NVIDIA Modulus
An NVIDIA AI Enterprise subscription is required to make the most of NVIDIA’s AI frameworks. Instead of assembling thousands of co-dependent libraries and APIs, AI Enterprise provides a full software stack and up to 24x7 technical support. AI Enterprise is licensed per GPU and is available on all AI systems from Scan.
Running NVIDIA Modulus software will require different hardware configurations for the development and training phases. Our health-check consultancy services verifies the suitability of your existing infrastructure to effectively run NVIDIA Modulus, with our experts offering advice on what upgrades to make if required.
AI Infrastructure for NVIDIA Modulus
Custom-built AI workstations from Scan 3XS Systems are the perfect way to start developing with NVIDIA Modulus. Workstations can be configured with up to six NVIDIA RTX GPUs and are fine-tuned by our hardware engineers for performance, reliability and low-noise. The custom software stack includes NVIDIA Modulus, Ubuntu operating system and Docker for containerised workloads.
Custom-built AI servers from Scan 3XS Systems and NVIDIA DGX appliances, combined with PEAK:AIO software defined storage servers are the perfect way to train with NVIDIA Modulus. These systems can be configured with up to eight NVIDIA GPUs and are fine-tuned by our hardware engineers for performance, reliability and low-noise. The custom software stack includes NVIDIA Modulus, operating system and management tools.
NVIDIA Modulus projects can also be developed, trained and scaled in a virtual environment using the Scan AI & Compute Cloud. This on-demand service has been developed from the ground up for intensive GPU-accelerated workloads and offers bespoke cloud instances to suit your individual projects, that can flex as your requirements develop and evolve.
Get the most from your AI Infrastructure
Ensuring your NVIDIA Modulus infrastructure remains at its optimum is key to your applications continued evolution and expansion. The Scan AI Ecosystem includes a range of professional services including installation, configuration and monitoring alongside consulting for cyber security and end-to-end project management.
If necessary, we can arrange hosting of your AI infrastructure with a range of datacentre partners, based either in the UK or in a variety of Scandinavian countries to take advantage of renewable energy sources. We also provide colocation and relocation services to best suit your business and data compliance needs.
The nature of energy production in the UK often results in surplus renewable power being stranded in between the wind farms and the grid. This energy can be utilised by modular datacentres located where the surplus energy is generated, unlocking low-cost compute power and reduced costs compared to building a new permanent datacentre.
Share your NVIDIA Modulus Success
Partnering with us for a case study increases brand exposure through coordinated joint-marketing with Scan and NVIDIA.
VIEW OUR CASE STUDIES >Get Started with NVIDIA Modulus
What is NVIDIA TAO Toolkit?
Use the NVIDIA TAO Toolkit open source framework to enhance your machine vision applications. TAO Toolkit puts the power of Vision Transformers in the hands of your developers, eliminating the need for mountains of data and an army of data scientists as you create AI/machine learning models and speed up the development process with transfer learning. TAO Toolkit, built on TensorFlow and PyTorch, uses the power of transfer learning while simultaneously simplifying the model training process and optimising the model for inference throughput on practically any platform.
Is NVIDIA TAO Toolkit Right for You?
To ensure NVIDIA TAO Toolkit is the right application for your datasets and challenges, Scan AI offers a free guided proof of concept (POC) trial. Our cloud-based GPU-accelerated hardware is located in UK datacentres and can be accessed securely from anywhere.
You are able to use your own data and will be supported by our expert data scientists to ensure you get the most from trying NVIDIA TAO Toolkit.
Leverage our Expertise
After your POC, our in-house team of data scientists and developers can identify and extract value from your data, speeding up application deployment.
Our team will work to provide analytical insight from your structured and unstructured data, defining a clear strategy which will be presented to your organisation, identifying quick wins, potential risks and include suggestions on how to minimise bottlenecks. Consultancy of this type is often pivotal in shaping your hardware plans too, as data driven projects always offer clearer system requirements when choosing AI infrastructure optimised for NVIDIA TAO Toolkit.
Additionally, we will train your teams to expand data science knowledge within your organisation.
Further your Knowledge
Scan AI is a certified NVIDIA Deep Learning Institute (DLI) provider. We offer a wide range of instructor-led courses to help your organisation get the most from GPU-accelerated hardware.
NVIDIA TAO Toolkit physics-based models will scale rapidly, so the ‘Data Parallelism: How to Train Deep Learning Models on Multiple GPUs’ course is ideal to expand your understanding and prepare for your projects.
Get ready for NVIDIA TAO Toolkit
An NVIDIA AI Enterprise subscription is required to make the most of NVIDIA’s AI frameworks. Instead of assembling thousands of co-dependent libraries and APIs, AI Enterprise provides a full software stack and up to 24x7 technical support. AI Enterprise is licensed per GPU and is available on all AI systems from Scan.
Running NVIDIA TAO Toolkit software will require different hardware configurations for the development and training phases. Our health-check consultancy services verifies the suitability of your existing infrastructure to effectively run NVIDIA ACE, with our experts offering advice on what upgrades to make if required.
AI Infrastructure for NVIDIA TAO Toolkit
Custom-built AI workstations from Scan 3XS Systems are the perfect way to start developing with NVIDIA TAO Toolkit. Workstations can be configured with up to six NVIDIA RTX GPUs and are fine-tuned by our hardware engineers for performance, reliability and low-noise. The custom software stack includes NVIDIA TAO Toolkit, Ubuntu operating system and Docker for containerised workloads.
Custom-built AI servers from Scan 3XS Systems and NVIDIA DGX appliances, combined with PEAK:AIO software defined storage servers are the perfect way to train with NVIDIA ACE. These systems can be configured with up to eight NVIDIA GPUs and are fine-tuned by our hardware engineers for performance, reliability and low-noise. The custom software stack includes NVIDIA Morpheus, operating system and management tools.
NVIDIA TAO Toolkit projects can also be developed, trained and scaled in a virtual environment using the Scan AI & Compute Cloud. This on-demand service has been developed from the ground up for intensive GPU-accelerated workloads and offers bespoke cloud instances to suit your individual projects, that can flex as your requirements develop and evolve.
Get the most from your AI Infrastructure
Ensuring your NVIDIA TAO Toolkit infrastructure remains at its optimum is key to your applications continued evolution and expansion. The Scan AI Ecosystem includes a range of professional services including installation, configuration and monitoring alongside consulting for cyber security and end-to-end project management.
If necessary, we can arrange hosting of your AI infrastructure with a range of datacentre partners, based either in the UK or in a variety of Scandinavian countries to take advantage of renewable energy sources. We also provide colocation and relocation services to best suit your business and data compliance needs.
The nature of energy production in the UK often results in surplus renewable power being stranded in between the wind farms and the grid. This energy can be utilised by modular datacentres located where the surplus energy is generated, unlocking low-cost compute power and reduced costs compared to building a new permanent datacentre.
Share your NVIDIA TAO Toolkit Success
Partnering with us for a case study increases brand exposure through coordinated joint-marketing with Scan and NVIDIA.
VIEW OUR CASE STUDIES >Get Started with NVIDIA TAO Toolkit
What is NVIDIA Clara Discovery?
Use the NVIDIA Clara Discovery open-source framework to power AI solutions for drug discovery. Clara Discovery is a collection of frameworks, applications and AI models enabling GPU-accelerated computational drug discovery. It combines accelerated computing, AI and machine learning in genomics, proteomics, microscopy, virtual screening, computational chemistry, visualisation, clinical imaging and natural language processing. It is also complemented by NVIDIA Parabricks and NVIDIA BioNeMo.
Is NVIDIA Clara Discovery Right for You?
To ensure NVIDIA Clara Discovery is the right application for your datasets and challenges, Scan AI offers a free guided proof of concept (POC) trial. Our cloud-based GPU-accelerated hardware is located in UK datacentres and can be accessed securely from anywhere.
You are able to use your own data and will be supported by our expert data scientists to ensure you get the most from trying NVIDIA Clara Discovery.
Leverage our Expertise
After your POC, our in-house team of data scientists and developers can identify and extract value from your data, speeding up application deployment.
Our team will work to provide analytical insight from your structured and unstructured data, defining a clear strategy which will be presented to your organisation, identifying quick wins, potential risks and include suggestions on how to minimise bottlenecks. Consultancy of this type is often pivotal in shaping your hardware plans too, as data driven projects always offer clearer system requirements when choosing AI infrastructure optimised for NVIDIA Clara Discovery.
Additionally, we will train your teams to expand data science knowledge within your organisation.
Further your Knowledge
Scan AI is a certified NVIDIA Deep Learning Institute (DLI) provider. We offer a wide range of instructor-led courses to help your organisation get the most from GPU-accelerated hardware.
NVIDIA Clara Discovery physics-based models will scale rapidly, so the ‘Data Parallelism: How to Train Deep Learning Models on Multiple GPUs’ course is ideal to expand your understanding and prepare for your projects.
Get ready for NVIDIA Clara Discovery
An NVIDIA AI Enterprise subscription is required to make the most of NVIDIA’s AI frameworks. Instead of assembling thousands of co-dependent libraries and APIs, AI Enterprise provides a full software stack and up to 24x7 technical support. AI Enterprise is licensed per GPU and is available on all AI systems from Scan.
Running NVIDIA Clara Discovery software will require different hardware configurations for the development and training phases. Our health-check consultancy services verifies the suitability of your existing infrastructure to effectively run NVIDIA ACE, with our experts offering advice on what upgrades to make if required.
You may have suitable existing GPU resource to effectively run NVIDIA Clara Discovery, but installed across a variety of workstations and/or servers. Run:ai software creates a virtual GPU pool, enabling you to allocate the right amount of compute for every task; from fractions of a GPU, to multiple GPUs.
AI Infrastructure for NVIDIA Clara Discovery
Custom-built AI workstations from Scan 3XS Systems are the perfect way to start developing with NVIDIA Clara Discovery. Workstations can be configured with up to six NVIDIA RTX GPUs and are fine-tuned by our hardware engineers for performance, reliability and low-noise. The custom software stack includes NVIDIA Clara Discovery, Ubuntu operating system and Docker for containerised workloads.
Custom-built AI servers from Scan 3XS Systems and NVIDIA DGX appliances, combined with PEAK:AIO software defined storage servers are the perfect way to train with NVIDIA ACE. These systems can be configured with up to eight NVIDIA GPUs and are fine-tuned by our hardware engineers for performance, reliability and low-noise. The custom software stack includes NVIDIA Clara Discovery, operating system and management tools.
NVIDIA Clara Discovery projects can also be developed, trained and scaled in a virtual environment using the Scan AI & Compute Cloud. This on-demand service has been developed from the ground up for intensive GPU-accelerated workloads and offers bespoke cloud instances to suit your individual projects, that can flex as your requirements develop and evolve.
Get the most from your AI Infrastructure
Ensuring your NVIDIA Clara Discovery infrastructure remains at its optimum is key to your applications continued evolution and expansion. The Scan AI Ecosystem includes a range of professional services including installation, configuration and monitoring alongside consulting for cyber security and end-to-end project management.
If necessary, we can arrange hosting of your AI infrastructure with a range of datacentre partners, based either in the UK or in a variety of Scandinavian countries to take advantage of renewable energy sources. We also provide colocation and relocation services to best suit your business and data compliance needs.
The nature of energy production in the UK often results in surplus renewable power being stranded in between the wind farms and the grid. This energy can be utilised by modular datacentres located where the surplus energy is generated, unlocking low-cost compute power and reduced costs compared to building a new permanent datacentre.
Share your NVIDIA Clara Discovery Success
Partnering with us for a case study increases brand exposure through coordinated joint-marketing with Scan and NVIDIA.
VIEW OUR CASE STUDIES >Get Started with NVIDIA Clara Discovery
What is NVIDIA Omniverse?
Use the NVIDIA Omniverse platform of APIs, SDKs and services to enable the simple integration of Universal Scene Description (OpenUSD) and RTX rendering technologies into existing software tools and simulation workflows for building AI systems. Start developing custom applications and tools from scratch with Omniverse Kit SDK for local and virtual workstations, or deploy Omniverse Enterprise collaboration capability across your entire organisation.
Is NVIDIA Omniverse Right for You?
To ensure NVIDIA Omniverse is the right application for your datasets and challenges, Scan AI offers a free guided proof of concept (POC) trial. Our cloud-based GPU-accelerated hardware is located in UK datacentres and can be accessed securely from anywhere.
You are able to use your own data and will be supported by our expert data scientists to ensure you get the most from trying NVIDIA Omniverse.
Leverage our Expertise
After your POC, our in-house team of data scientists and developers can identify and extract value from your data, speeding up application deployment.
Our team will work to provide analytical insight from your structured and unstructured data, defining a clear strategy which will be presented to your organisation, identifying quick wins, potential risks and include suggestions on how to minimise bottlenecks. Consultancy of this type is often pivotal in shaping your hardware plans too, as data driven projects always offer clearer system requirements when choosing AI infrastructure optimised for NVIDIA Omniverse.
Additionally, we will train your teams to expand data science knowledge within your organisation.
Further your Knowledge
Scan AI is a certified NVIDIA Deep Learning Institute (DLI) provider. We offer a wide range of instructor-led courses to help your organisation get the most from GPU-accelerated hardware.
NVIDIA Omniverse physics-based models will scale rapidly, so the ‘Data Parallelism: How to Train Deep Learning Models on Multiple GPUs’ course is ideal to expand your understanding and prepare for your projects.
Get ready for NVIDIA Omniverse
An NVIDIA AI Enterprise subscription is required to make the most of NVIDIA’s AI frameworks. Instead of assembling thousands of co-dependent libraries and APIs, AI Enterprise provides a full software stack and up to 24x7 technical support. AI Enterprise is licensed per GPU and is available on all AI systems from Scan.
Running NVIDIA Omniverse software will require different hardware configurations for the development and training phases. Our health-check consultancy services verifies the suitability of your existing infrastructure to effectively run NVIDIA ACE, with our experts offering advice on what upgrades to make if required.
AI Infrastructure for NVIDIA Omniverse
Custom-built AI workstations from Scan 3XS Systems are the perfect way to start developing with NVIDIA Omniverse. Workstations can be configured with up to six NVIDIA RTX GPUs and are fine-tuned by our hardware engineers for performance, reliability and low-noise.
Custom-built AI servers from Scan 3XS Systems and NVIDIA DGX appliances, combined with PEAK:AIO software defined storage servers are the perfect way to train with NVIDIA ACE. These systems can be configured with up to eight NVIDIA GPUs and are fine-tuned by our hardware engineers for performance, reliability and low-noise. The custom software stack includes NVIDIA Omniverse, operating system and management tools.
NVIDIA Omniverse projects can also be developed, trained and scaled in a virtual environment using the Scan AI & Compute Cloud. This on-demand service has been developed from the ground up for intensive GPU-accelerated workloads and offers bespoke cloud instances to suit your individual projects, that can flex as your requirements develop and evolve.
Get the most from your AI Infrastructure
Ensuring your NVIDIA Omniverse infrastructure remains at its optimum is key to your applications continued evolution and expansion. The Scan AI Ecosystem includes a range of professional services including installation, configuration and monitoring alongside consulting for cyber security and end-to-end project management.
If necessary, we can arrange hosting of your AI infrastructure with a range of datacentre partners, based either in the UK or in a variety of Scandinavian countries to take advantage of renewable energy sources. We also provide colocation and relocation services to best suit your business and data compliance needs.
The nature of energy production in the UK often results in surplus renewable power being stranded in between the wind farms and the grid. This energy can be utilised by modular datacentres located where the surplus energy is generated, unlocking low-cost compute power and reduced costs compared to building a new permanent datacentre.
Share your NVIDIA Omniverse Success
Partnering with us for a case study increases brand exposure through coordinated joint-marketing with Scan and NVIDIA.
VIEW OUR CASE STUDIES >Get Started with NVIDIA Omniverse
What is MONAI?
Use the MONAI open-source framework to accelerate the pace of innovation and clinical translation by building a robust software framework that benefits nearly every level of medical imaging, deep learning research and deployment. MONAI is comprehensive suite of enterprise-grade containers, AI models and cloud APIs crafted to accelerate medical imaging workflows, helping provide developers with secure, NVIDIA-supported, state-of-the-art and domain-specific AI workflows.
Is NVIDIA MONAI Right for You?
To ensure NVIDIA MONAI is the right application for your datasets and challenges, Scan AI offers a free guided proof of concept (POC) trial. Our cloud-based GPU-accelerated hardware is located in UK datacentres and can be accessed securely from anywhere.
You are able to use your own data and will be supported by our expert data scientists to ensure you get the most from trying NVIDIA MONAI.
Leverage our Expertise
After your POC, our in-house team of data scientists and developers can identify and extract value from your data, speeding up application deployment.
Our team will work to provide analytical insight from your structured and unstructured data, defining a clear strategy which will be presented to your organisation, identifying quick wins, potential risks and include suggestions on how to minimise bottlenecks. Consultancy of this type is often pivotal in shaping your hardware plans too, as data driven projects always offer clearer system requirements when choosing AI infrastructure optimised for NVIDIA MONAI.
Additionally, we will train your teams to expand data science knowledge within your organisation.
Further your Knowledge
Scan AI is a certified NVIDIA Deep Learning Institute (DLI) provider. We offer a wide range of instructor-led courses to help your organisation get the most from GPU-accelerated hardware.
NVIDIA MONAI physics-based models will scale rapidly, so the ‘Data Parallelism: How to Train Deep Learning Models on Multiple GPUs’ course is ideal to expand your understanding and prepare for your projects.
Get ready for NVIDIA MONAI
An NVIDIA AI Enterprise subscription is required to make the most of NVIDIA’s AI frameworks. Instead of assembling thousands of co-dependent libraries and APIs, AI Enterprise provides a full software stack and up to 24x7 technical support. AI Enterprise is licensed per GPU and is available on all AI systems from Scan.
Running NVIDIA MONAI software will require different hardware configurations for the development and training phases. Our health-check consultancy services verifies the suitability of your existing infrastructure to effectively run NVIDIA ACE, with our experts offering advice on what upgrades to make if required.
AI Infrastructure for NVIDIA MONAI
Custom-built AI workstations from Scan 3XS Systems are the perfect way to start developing with NVIDIA MONAI. Workstations can be configured with up to six NVIDIA RTX GPUs and are fine-tuned by our hardware engineers for performance, reliability and low-noise. The custom software stack includes NVIDIA MONAI, Ubuntu operating system and Docker for containerised workloads.
Custom-built AI servers from Scan 3XS Systems and NVIDIA DGX appliances, combined with PEAK:AIO software defined storage servers are the perfect way to train with NVIDIA ACE. These systems can be configured with up to eight NVIDIA GPUs and are fine-tuned by our hardware engineers for performance, reliability and low-noise. The custom software stack includes NVIDIA MONAI, operating system and management tools.
NVIDIA MONAI projects can also be developed, trained and scaled in a virtual environment using the Scan AI & Compute Cloud. This on-demand service has been developed from the ground up for intensive GPU-accelerated workloads and offers bespoke cloud instances to suit your individual projects, that can flex as your requirements develop and evolve.
Get the most from your AI Infrastructure
Ensuring your NVIDIA MONAI infrastructure remains at its optimum is key to your applications continued evolution and expansion. The Scan AI Ecosystem includes a range of professional services including installation, configuration and monitoring alongside consulting for cyber security and end-to-end project management.
If necessary, we can arrange hosting of your AI infrastructure with a range of datacentre partners, based either in the UK or in a variety of Scandinavian countries to take advantage of renewable energy sources. We also provide colocation and relocation services to best suit your business and data compliance needs.
The nature of energy production in the UK often results in surplus renewable power being stranded in between the wind farms and the grid. This energy can be utilised by modular datacentres located where the surplus energy is generated, unlocking low-cost compute power and reduced costs compared to building a new permanent datacentre.
Share your NVIDIA MONAI Success
Partnering with us for a case study increases brand exposure through coordinated joint-marketing with Scan and NVIDIA.
VIEW OUR CASE STUDIES >Get Started with NVIDIA MONAI
What is NVIDIA BioNeMo?
Use the NVIDIA BioNeMo open-source framework to power drug discovery that simplifies and accelerates model training. BioNeMo builds on NVIDIA NeMo capabilities using LLMs and generative AI to offer the quickest path to AI-powered drug discovery. By taking the hassle out of managing AI supercomputing resources, generative AI for drug discovery can scale effortlessly, whether running in the cloud or on-premise systems.
Is NVIDIA BioNeMo Right for You?
To ensure NVIDIA BioNeMo is the right application for your datasets and challenges, Scan AI offers a free guided proof of concept (POC) trial. Our cloud-based GPU-accelerated hardware is located in UK datacentres and can be accessed securely from anywhere.
You are able to use your own data and will be supported by our expert data scientists to ensure you get the most from trying NVIDIA BioNeMo.
Leverage our Expertise
After your POC, our in-house team of data scientists and developers can identify and extract value from your data, speeding up application deployment.
Our team will work to provide analytical insight from your structured and unstructured data, defining a clear strategy which will be presented to your organisation, identifying quick wins, potential risks and include suggestions on how to minimise bottlenecks. Consultancy of this type is often pivotal in shaping your hardware plans too, as data driven projects always offer clearer system requirements when choosing AI infrastructure optimised for NVIDIA BioNeMo.
Additionally, we will train your teams to expand data science knowledge within your organisation.
Further your Knowledge
Scan AI is a certified NVIDIA Deep Learning Institute (DLI) provider. We offer a wide range of instructor-led courses to help your organisation get the most from GPU-accelerated hardware.
NVIDIA BioNeMo physics-based models will scale rapidly, so the ‘Data Parallelism: How to Train Deep Learning Models on Multiple GPUs’ course is ideal to expand your understanding and prepare for your projects.
Get ready for NVIDIA BioNeMo
An NVIDIA AI Enterprise subscription is required to make the most of NVIDIA’s AI frameworks. Instead of assembling thousands of co-dependent libraries and APIs, AI Enterprise provides a full software stack and up to 24x7 technical support. AI Enterprise is licensed per GPU and is available on all AI systems from Scan.
Running NVIDIA BioNeMo software will require different hardware configurations for the development and training phases. Our health-check consultancy services verifies the suitability of your existing infrastructure to effectively run NVIDIA BioNeMo, with our experts offering advice on what upgrades to make if required.
AI Infrastructure for NVIDIA BioNeMo
Custom-built AI workstations from Scan 3XS Systems are the perfect way to start developing with NVIDIA BioNeMo. Workstations can be configured with up to six NVIDIA RTX GPUs and are fine-tuned by our hardware engineers for performance, reliability and low-noise. The custom software stack includes NVIDIA BioNeMo, Ubuntu operating system and Docker for containerised workloads.
Custom-built AI servers from Scan 3XS Systems and NVIDIA DGX appliances, combined with PEAK:AIO software defined storage servers are the perfect way to train with NVIDIA ACE. These systems can be configured with up to eight NVIDIA GPUs and are fine-tuned by our hardware engineers for performance, reliability and low-noise. The custom software stack includes NVIDIA BioNeMo, operating system and management tools.
NVIDIA BioNeMo projects can also be developed, trained and scaled in a virtual environment using the Scan AI & Compute Cloud. This on-demand service has been developed from the ground up for intensive GPU-accelerated workloads and offers bespoke cloud instances to suit your individual projects, that can flex as your requirements develop and evolve.
Get the most from your AI Infrastructure
Ensuring your NVIDIA BioNeMo infrastructure remains at its optimum is key to your applications continued evolution and expansion. The Scan AI Ecosystem includes a range of professional services including installation, configuration and monitoring alongside consulting for cyber security and end-to-end project management.
If necessary, we can arrange hosting of your AI infrastructure with a range of datacentre partners, based either in the UK or in a variety of Scandinavian countries to take advantage of renewable energy sources. We also provide colocation and relocation services to best suit your business and data compliance needs.
The nature of energy production in the UK often results in surplus renewable power being stranded in between the wind farms and the grid. This energy can be utilised by modular datacentres located where the surplus energy is generated, unlocking low-cost compute power and reduced costs compared to building a new permanent datacentre.
Share your NVIDIA BioNeMo Success
Partnering with us for a case study increases brand exposure through coordinated joint-marketing with Scan and NVIDIA.
VIEW OUR CASE STUDIES >Get Started with NVIDIA BioNeMo
What is NVIDIA Isaac?
Use the NVIDIA Isaac open source framework to power robotics research and development including machine vision, manipulation and simulation. The Isaac platform, hosted in Omniverse and supported by TAO Toolkit, gives you a faster, better way to design, test and train AI-based robots, to deliver scalable, photorealistic and physically accurate virtual environments for building high-fidelity simulations.
Is NVIDIA Isaac Right for You?
To ensure NVIDIA Isaac is the right application for your datasets and challenges, Scan AI offers a free guided proof of concept (POC) trial. Our cloud-based GPU-accelerated hardware is located in UK datacentres and can be accessed securely from anywhere.
You are able to use your own data and will be supported by our expert data scientists to ensure you get the most from trying NVIDIA Isaac.
Leverage our Expertise
After your POC, our in-house team of data scientists and developers can identify and extract value from your data, speeding up application deployment.
Our team will work to provide analytical insight from your structured and unstructured data, defining a clear strategy which will be presented to your organisation, identifying quick wins, potential risks and include suggestions on how to minimise bottlenecks. Consultancy of this type is often pivotal in shaping your hardware plans too, as data driven projects always offer clearer system requirements when choosing AI infrastructure optimised for NVIDIA Isaac.
Additionally, we will train your teams to expand data science knowledge within your organisation.
Further your Knowledge
Scan AI is a certified NVIDIA Deep Learning Institute (DLI) provider. We offer a wide range of instructor-led courses to help your organisation get the most from GPU-accelerated hardware.
NVIDIA Isaac physics-based models will scale rapidly, so the ‘Data Parallelism: How to Train Deep Learning Models on Multiple GPUs’ course is ideal to expand your understanding and prepare for your projects.
Get ready for NVIDIA Isaac
An NVIDIA AI Enterprise subscription is required to make the most of NVIDIA’s AI frameworks. Instead of assembling thousands of co-dependent libraries and APIs, AI Enterprise provides a full software stack and up to 24x7 technical support. AI Enterprise is licensed per GPU and is available on all AI systems from Scan.
Running NVIDIA Isaac software will require different hardware configurations for the development and training phases. Our health-check consultancy services verifies the suitability of your existing infrastructure to effectively run NVIDIA BioNeMo, with our experts offering advice on what upgrades to make if required.
AI Infrastructure for NVIDIA Isaac
Custom-built AI workstations from Scan 3XS Systems are the perfect way to start developing with NVIDIA Isaac. Workstations can be configured with up to six NVIDIA RTX GPUs and are fine-tuned by our hardware engineers for performance, reliability and low-noise. The custom software stack includes NVIDIA Isaac, Ubuntu operating system and Docker for containerised workloads.
Custom-built AI servers from Scan 3XS Systems and NVIDIA DGX appliances, combined with PEAK:AIO software defined storage servers are the perfect way to train with NVIDIA ACE. These systems can be configured with up to eight NVIDIA GPUs and are fine-tuned by our hardware engineers for performance, reliability and low-noise. The custom software stack includes NVIDIA Isaac, operating system and management tools.
NVIDIA Isaac projects can also be developed, trained and scaled in a virtual environment using the Scan AI & Compute Cloud. This on-demand service has been developed from the ground up for intensive GPU-accelerated workloads and offers bespoke cloud instances to suit your individual projects, that can flex as your requirements develop and evolve.
Get the most from your AI Infrastructure
Ensuring your NVIDIA Isaac infrastructure remains at its optimum is key to your applications continued evolution and expansion. The Scan AI Ecosystem includes a range of professional services including installation, configuration and monitoring alongside consulting for cyber security and end-to-end project management.
If necessary, we can arrange hosting of your AI infrastructure with a range of datacentre partners, based either in the UK or in a variety of Scandinavian countries to take advantage of renewable energy sources. We also provide colocation and relocation services to best suit your business and data compliance needs.
The nature of energy production in the UK often results in surplus renewable power being stranded in between the wind farms and the grid. This energy can be utilised by modular datacentres located where the surplus energy is generated, unlocking low-cost compute power and reduced costs compared to building a new permanent datacentre.
Share your NVIDIA Isaac Success
Partnering with us for a case study increases brand exposure through coordinated joint-marketing with Scan and NVIDIA.
VIEW OUR CASE STUDIES >Get Started with NVIDIA Isaac
What is NVIDIA Merlin?
Use the NVIDIA Merlin open source framework to build high-performing recommender systems at scale. Merlin addresses common preprocessing, feature engineering, training, inferencing and deployment challenges, and is optimised to support the retrieval, filtering, scoring and ordering of hundreds of terabytes of data, all accessible through easy-to-use APIs. With Merlin, better predictions, increased click-through rates, and faster deployment to production are within reach, supported by NVIDIA RAPIDS and NVIDIA Triton.
Is NVIDIA Merlin Right for You?
To ensure NVIDIA Merlin is the right application for your datasets and challenges, Scan AI offers a free guided proof of concept (POC) trial. Our cloud-based GPU-accelerated hardware is located in UK datacentres and can be accessed securely from anywhere.
You are able to use your own data and will be supported by our expert data scientists to ensure you get the most from trying NVIDIA Merlin.
Leverage our Expertise
After your POC, our in-house team of data scientists and developers can identify and extract value from your data, speeding up application deployment.
Our team will work to provide analytical insight from your structured and unstructured data, defining a clear strategy which will be presented to your organisation, identifying quick wins, potential risks and include suggestions on how to minimise bottlenecks. Consultancy of this type is often pivotal in shaping your hardware plans too, as data driven projects always offer clearer system requirements when choosing AI infrastructure optimised for NVIDIA Merlin.
Additionally, we will train your teams to expand data science knowledge within your organisation.
Further your Knowledge
Scan AI is a certified NVIDIA Deep Learning Institute (DLI) provider. We offer a wide range of instructor-led courses to help your organisation get the most from GPU-accelerated hardware.
NVIDIA Merlin physics-based models will scale rapidly, so the ‘Data Parallelism: How to Train Deep Learning Models on Multiple GPUs’ course is ideal to expand your understanding and prepare for your projects.
Get ready for NVIDIA Merlin
An NVIDIA AI Enterprise subscription is required to make the most of NVIDIA’s AI frameworks. Instead of assembling thousands of co-dependent libraries and APIs, AI Enterprise provides a full software stack and up to 24x7 technical support. AI Enterprise is licensed per GPU and is available on all AI systems from Scan.
Running NVIDIA Merlin software will require different hardware configurations for the development and training phases. Our health-check consultancy services verifies the suitability of your existing infrastructure to effectively run NVIDIA BioNeMo, with our experts offering advice on what upgrades to make if required.
AI Infrastructure for NVIDIA Merlin
Custom-built AI workstations from Scan 3XS Systems are the perfect way to start developing with NVIDIA Merlin. Workstations can be configured with up to six NVIDIA RTX GPUs and are fine-tuned by our hardware engineers for performance, reliability and low-noise. The custom software stack includes NVIDIA Merlin, Ubuntu operating system and Docker for containerised workloads.
Custom-built AI servers from Scan 3XS Systems and NVIDIA DGX appliances, combined with PEAK:AIO software defined storage servers are the perfect way to train with NVIDIA ACE. These systems can be configured with up to eight NVIDIA GPUs and are fine-tuned by our hardware engineers for performance, reliability and low-noise. The custom software stack includes NVIDIA Merlin, operating system and management tools.
NVIDIA Merlin projects can also be developed, trained and scaled in a virtual environment using the Scan AI & Compute Cloud. This on-demand service has been developed from the ground up for intensive GPU-accelerated workloads and offers bespoke cloud instances to suit your individual projects, that can flex as your requirements develop and evolve.
Get the most from your AI Infrastructure
Ensuring your NVIDIA Merlin infrastructure remains at its optimum is key to your applications continued evolution and expansion. The Scan AI Ecosystem includes a range of professional services including installation, configuration and monitoring alongside consulting for cyber security and end-to-end project management.
If necessary, we can arrange hosting of your AI infrastructure with a range of datacentre partners, based either in the UK or in a variety of Scandinavian countries to take advantage of renewable energy sources. We also provide colocation and relocation services to best suit your business and data compliance needs.
The nature of energy production in the UK often results in surplus renewable power being stranded in between the wind farms and the grid. This energy can be utilised by modular datacentres located where the surplus energy is generated, unlocking low-cost compute power and reduced costs compared to building a new permanent datacentre.
Share your NVIDIA Merlin Success
Partnering with us for a case study increases brand exposure through coordinated joint-marketing with Scan and NVIDIA.
VIEW OUR CASE STUDIES >Get Started with NVIDIA Merlin
What is NVIDIA NeMo?
Use the NVIDIA NeMo open-source framework to power the development of custom LLMs and generative AI models. The NeMo framework is designed for enterprise development, and is an end-to-end, cloud-native framework for curating data, training and customising foundation models, and running inference at scale. It supports text-to-text, text-to-image, and text-to-3D models and image-to-image generation.
A second framework, NVIDIA BioNeMo, expands NeMo’s capabilities into scientific applications of LLMs for the pharmaceutical and biotechnology industries.
Is NVIDIA NeMo Right for You?
To ensure NVIDIA NeMo is the right application for your datasets and challenges, Scan AI offers a free guided proof of concept (POC) trial. Our cloud-based GPU-accelerated hardware is located in UK datacentres and can be accessed securely from anywhere.
You are able to use your own data and will be supported by our expert data scientists to ensure you get the most from trying NVIDIA NeMo.
Leverage our Expertise
After your POC, our in-house team of data scientists and developers can identify and extract value from your data, speeding up application deployment.
Our team will work to provide analytical insight from your structured and unstructured data, defining a clear strategy which will be presented to your organisation, identifying quick wins, potential risks and include suggestions on how to minimise bottlenecks. Consultancy of this type is often pivotal in shaping your hardware plans too, as data driven projects always offer clearer system requirements when choosing AI infrastructure optimised for NVIDIA NeMo.
Additionally, we will train your teams to expand data science knowledge within your organisation.
Further your Knowledge
Scan AI is a certified NVIDIA Deep Learning Institute (DLI) provider. We offer a wide range of instructor-led courses to help your organisation get the most from GPU-accelerated hardware.
NVIDIA NeMo physics-based models will scale rapidly, so the ‘Data Parallelism: How to Train Deep Learning Models on Multiple GPUs’ course is ideal to expand your understanding and prepare for your projects.
Get ready for NVIDIA NeMo
An NVIDIA AI Enterprise subscription is required to make the most of NVIDIA’s AI frameworks. Instead of assembling thousands of co-dependent libraries and APIs, AI Enterprise provides a full software stack and up to 24x7 technical support. AI Enterprise is licensed per GPU and is available on all AI systems from Scan.
Running NVIDIA NeMo software will require different hardware configurations for the development and training phases. Our health-check consultancy services verifies the suitability of your existing infrastructure to effectively run NVIDIA BioNeMo, with our experts offering advice on what upgrades to make if required.
AI Infrastructure for NVIDIA NeMo
Custom-built AI workstations from Scan 3XS Systems are the perfect way to start developing with NVIDIA NeMo. Workstations can be configured with up to six NVIDIA RTX GPUs and are fine-tuned by our hardware engineers for performance, reliability and low-noise. The custom software stack includes NVIDIA NeMo, Ubuntu operating system and Docker for containerised workloads.
Custom-built AI servers from Scan 3XS Systems and NVIDIA DGX appliances, combined with PEAK:AIO software defined storage servers are the perfect way to train with NVIDIA ACE. These systems can be configured with up to eight NVIDIA GPUs and are fine-tuned by our hardware engineers for performance, reliability and low-noise. The custom software stack includes NVIDIA NeMo, operating system and management tools.
NVIDIA NeMo projects can also be developed, trained and scaled in a virtual environment using the Scan AI & Compute Cloud. This on-demand service has been developed from the ground up for intensive GPU-accelerated workloads and offers bespoke cloud instances to suit your individual projects, that can flex as your requirements develop and evolve.
Get the most from your AI Infrastructure
Ensuring your NVIDIA NeMo infrastructure remains at its optimum is key to your applications continued evolution and expansion. The Scan AI Ecosystem includes a range of professional services including installation, configuration and monitoring alongside consulting for cyber security and end-to-end project management.
If necessary, we can arrange hosting of your AI infrastructure with a range of datacentre partners, based either in the UK or in a variety of Scandinavian countries to take advantage of renewable energy sources. We also provide colocation and relocation services to best suit your business and data compliance needs.
The nature of energy production in the UK often results in surplus renewable power being stranded in between the wind farms and the grid. This energy can be utilised by modular datacentres located where the surplus energy is generated, unlocking low-cost compute power and reduced costs compared to building a new permanent datacentre.
Share your NVIDIA NeMo Success
Partnering with us for a case study increases brand exposure through coordinated joint-marketing with Scan and NVIDIA.
VIEW OUR CASE STUDIES >Get Started with NVIDIA NeMo
What is NVIDIA Triton?
Use the NVIDIA Triton open-source framework to power inference for every AI workload. Triton Inference Server acts to standardise AI model deployment and execution across a wide range of AI libraries including TensorFlow, PyTorch, Python, ONNX, NVIDIA TensorRT and RAPIDS. Triton also offers low latency and high throughput for large language model (LLM) inferencing, and provides a cloud and edge inferencing solution optimised for both CPUs and GPUs.
Is NVIDIA Triton Right for You?
To ensure NVIDIA Triton is the right application for your datasets and challenges, Scan AI offers a free guided proof of concept (POC) trial. Our cloud-based GPU-accelerated hardware is located in UK datacentres and can be accessed securely from anywhere.
You are able to use your own data and will be supported by our expert data scientists to ensure you get the most from trying NVIDIA Triton.
Leverage our Expertise
After your POC, our in-house team of data scientists and developers can identify and extract value from your data, speeding up application deployment.
Our team will work to provide analytical insight from your structured and unstructured data, defining a clear strategy which will be presented to your organisation, identifying quick wins, potential risks and include suggestions on how to minimise bottlenecks. Consultancy of this type is often pivotal in shaping your hardware plans too, as data driven projects always offer clearer system requirements when choosing AI infrastructure optimised for NVIDIA Triton.
Additionally, we will train your teams to expand data science knowledge within your organisation.
Further your Knowledge
Scan AI is a certified NVIDIA Deep Learning Institute (DLI) provider. We offer a wide range of instructor-led courses to help your organisation get the most from GPU-accelerated hardware.
NVIDIA Triton physics-based models will scale rapidly, so the ‘Data Parallelism: How to Train Deep Learning Models on Multiple GPUs’ course is ideal to expand your understanding and prepare for your projects.
Get ready for NVIDIA Triton
An NVIDIA AI Enterprise subscription is required to make the most of NVIDIA’s AI frameworks. Instead of assembling thousands of co-dependent libraries and APIs, AI Enterprise provides a full software stack and up to 24x7 technical support. AI Enterprise is licensed per GPU and is available on all AI systems from Scan.
Running NVIDIA Triton software will require different hardware configurations for the development and training phases. Our health-check consultancy services verifies the suitability of your existing infrastructure to effectively run NVIDIA BioNeMo, with our experts offering advice on what upgrades to make if required.
AI Infrastructure for NVIDIA Triton
Custom-built AI workstations from Scan 3XS Systems are the perfect way to start developing with NVIDIA Triton. Workstations can be configured with up to six NVIDIA RTX GPUs and are fine-tuned by our hardware engineers for performance, reliability and low-noise. The custom software stack includes NVIDIA Triton, Ubuntu operating system and Docker for containerised workloads.
Custom-built AI servers from Scan 3XS Systems and NVIDIA DGX appliances, combined with PEAK:AIO software defined storage servers are the perfect way to train with NVIDIA ACE. These systems can be configured with up to eight NVIDIA GPUs and are fine-tuned by our hardware engineers for performance, reliability and low-noise. The custom software stack includes NVIDIA Triton, operating system and management tools.
NVIDIA Triton projects can also be developed, trained and scaled in a virtual environment using the Scan AI & Compute Cloud. This on-demand service has been developed from the ground up for intensive GPU-accelerated workloads and offers bespoke cloud instances to suit your individual projects, that can flex as your requirements develop and evolve.
Get the most from your AI Infrastructure
Ensuring your NVIDIA Triton infrastructure remains at its optimum is key to your applications continued evolution and expansion. The Scan AI Ecosystem includes a range of professional services including installation, configuration and monitoring alongside consulting for cyber security and end-to-end project management.
If necessary, we can arrange hosting of your AI infrastructure with a range of datacentre partners, based either in the UK or in a variety of Scandinavian countries to take advantage of renewable energy sources. We also provide colocation and relocation services to best suit your business and data compliance needs.
The nature of energy production in the UK often results in surplus renewable power being stranded in between the wind farms and the grid. This energy can be utilised by modular datacentres located where the surplus energy is generated, unlocking low-cost compute power and reduced costs compared to building a new permanent datacentre.
Share your NVIDIA Triton Success
Partnering with us for a case study increases brand exposure through coordinated joint-marketing with Scan and NVIDIA.
VIEW OUR CASE STUDIES >Get Started with NVIDIA Triton
What is NVIDIA Holoscan?
Use the NVIDIA Holoscan open-source framework to power the accelerated, full-stack infrastructure required for scalable, software-defined, real-time processing of streaming medical data at the clinical edge. With Holoscan, developers can build medical devices that take AI applications directly to the operating room, processing streaming data from sensors for AI inference that helps clinical teams make patient-specific decisions and recommendations.
Is NVIDIA Holoscan Right for You?
To ensure NVIDIA Holoscan is the right application for your datasets and challenges, Scan AI offers a free guided proof of concept (POC) trial. Our cloud-based GPU-accelerated hardware is located in UK datacentres and can be accessed securely from anywhere.
You are able to use your own data and will be supported by our expert data scientists to ensure you get the most from trying NVIDIA Holoscan.
Leverage our Expertise
After your POC, our in-house team of data scientists and developers can identify and extract value from your data, speeding up application deployment.
Our team will work to provide analytical insight from your structured and unstructured data, defining a clear strategy which will be presented to your organisation, identifying quick wins, potential risks and include suggestions on how to minimise bottlenecks. Consultancy of this type is often pivotal in shaping your hardware plans too, as data driven projects always offer clearer system requirements when choosing AI infrastructure optimised for NVIDIA Holoscan.
Additionally, we will train your teams to expand data science knowledge within your organisation.
Further your Knowledge
Scan AI is a certified NVIDIA Deep Learning Institute (DLI) provider. We offer a wide range of instructor-led courses to help your organisation get the most from GPU-accelerated hardware.
NVIDIA Holoscan physics-based models will scale rapidly, so the ‘Data Parallelism: How to Train Deep Learning Models on Multiple GPUs’ course is ideal to expand your understanding and prepare for your projects.
Get ready for NVIDIA Holoscan
An NVIDIA AI Enterprise subscription is required to make the most of NVIDIA’s AI frameworks. Instead of assembling thousands of co-dependent libraries and APIs, AI Enterprise provides a full software stack and up to 24x7 technical support. AI Enterprise is licensed per GPU and is available on all AI systems from Scan.
Running NVIDIA Holoscan software will require different hardware configurations for the development and training phases. Our health-check consultancy services verifies the suitability of your existing infrastructure to effectively run NVIDIA BioNeMo, with our experts offering advice on what upgrades to make if required.
AI Infrastructure for NVIDIA Holoscan
Custom-built AI workstations from Scan 3XS Systems are the perfect way to start developing with NVIDIA Holoscan. Workstations can be configured with up to six NVIDIA RTX GPUs and are fine-tuned by our hardware engineers for performance, reliability and low-noise. The custom software stack includes NVIDIA Holoscan, Ubuntu operating system and Docker for containerised workloads.
Custom-built AI servers from Scan 3XS Systems and NVIDIA DGX appliances, combined with PEAK:AIO software defined storage servers are the perfect way to train with NVIDIA ACE. These systems can be configured with up to eight NVIDIA GPUs and are fine-tuned by our hardware engineers for performance, reliability and low-noise. The custom software stack includes NVIDIA Holoscan, operating system and management tools.
NVIDIA Holoscan projects can also be developed, trained and scaled in a virtual environment using the Scan AI & Compute Cloud. This on-demand service has been developed from the ground up for intensive GPU-accelerated workloads and offers bespoke cloud instances to suit your individual projects, that can flex as your requirements develop and evolve.
Get the most from your AI Infrastructure
Ensuring your NVIDIA Holoscan infrastructure remains at its optimum is key to your applications continued evolution and expansion. The Scan AI Ecosystem includes a range of professional services including installation, configuration and monitoring alongside consulting for cyber security and end-to-end project management.
If necessary, we can arrange hosting of your AI infrastructure with a range of datacentre partners, based either in the UK or in a variety of Scandinavian countries to take advantage of renewable energy sources. We also provide colocation and relocation services to best suit your business and data compliance needs.
The nature of energy production in the UK often results in surplus renewable power being stranded in between the wind farms and the grid. This energy can be utilised by modular datacentres located where the surplus energy is generated, unlocking low-cost compute power and reduced costs compared to building a new permanent datacentre.
Share your NVIDIA Holoscan Success
Partnering with us for a case study increases brand exposure through coordinated joint-marketing with Scan and NVIDIA.
VIEW OUR CASE STUDIES >Get Started with NVIDIA Holoscan
What is NVIDIA Clara Guardian?
Use the NVIDIA Clara Guardian open-source framework to simplify the development and deployment of smart sensors with multimodal AI, anywhere in a healthcare facility. It is a software suite for industry-standard next generation sequencing analysis on NVIDIA GPUs, consisting of everyday bioinformatics command-line tools running at blazing speeds. It is also complemented by Triton, TAO, Riva and NeMo frameworks.
Is NVIDIA Clara Guardian Right for You?
To ensure NVIDIA Clara Guardian is the right application for your datasets and challenges, Scan AI offers a free guided proof of concept (POC) trial. Our cloud-based GPU-accelerated hardware is located in UK datacentres and can be accessed securely from anywhere.
You are able to use your own data and will be supported by our expert data scientists to ensure you get the most from trying NVIDIA Clara Guardian.
Leverage our Expertise
After your POC, our in-house team of data scientists and developers can identify and extract value from your data, speeding up application deployment.
Our team will work to provide analytical insight from your structured and unstructured data, defining a clear strategy which will be presented to your organisation, identifying quick wins, potential risks and include suggestions on how to minimise bottlenecks. Consultancy of this type is often pivotal in shaping your hardware plans too, as data driven projects always offer clearer system requirements when choosing AI infrastructure optimised for NVIDIA Clara Guardian.
Additionally, we will train your teams to expand data science knowledge within your organisation.
Further your Knowledge
Scan AI is a certified NVIDIA Deep Learning Institute (DLI) provider. We offer a wide range of instructor-led courses to help your organisation get the most from GPU-accelerated hardware.
NVIDIA Clara Guardian physics-based models will scale rapidly, so the ‘Data Parallelism: How to Train Deep Learning Models on Multiple GPUs’ course is ideal to expand your understanding and prepare for your projects.
Get ready for NVIDIA Clara Guardian
An NVIDIA AI Enterprise subscription is required to make the most of NVIDIA’s AI frameworks. Instead of assembling thousands of co-dependent libraries and APIs, AI Enterprise provides a full software stack and up to 24x7 technical support. AI Enterprise is licensed per GPU and is available on all AI systems from Scan.
Running NVIDIA Clara Guardian software will require different hardware configurations for the development and training phases. Our health-check consultancy services verifies the suitability of your existing infrastructure to effectively run NVIDIA BioNeMo, with our experts offering advice on what upgrades to make if required.
You may have suitable existing GPU resource to effectively run NVIDIA Clara Guardian, but installed across a variety of workstations and/or servers. Run:ai software creates a virtual GPU pool, enabling you to allocate the right amount of compute for every task; from fractions of a GPU, to multiple GPUs.
AI Infrastructure for NVIDIA Clara Guardian
Custom-built AI workstations from Scan 3XS Systems are the perfect way to start developing with NVIDIA Clara Guardian. Workstations can be configured with up to six NVIDIA RTX GPUs and are fine-tuned by our hardware engineers for performance, reliability and low-noise. The custom software stack includes NVIDIA Clara Guardian, Ubuntu operating system and Docker for containerised workloads.
Custom-built AI servers from Scan 3XS Systems and NVIDIA DGX appliances, combined with PEAK:AIO software defined storage servers are the perfect way to train with NVIDIA ACE. These systems can be configured with up to eight NVIDIA GPUs and are fine-tuned by our hardware engineers for performance, reliability and low-noise. The custom software stack includes NVIDIA Clara Guardian, operating system and management tools.
NVIDIA Clara Guardian projects can also be developed, trained and scaled in a virtual environment using the Scan AI & Compute Cloud. This on-demand service has been developed from the ground up for intensive GPU-accelerated workloads and offers bespoke cloud instances to suit your individual projects, that can flex as your requirements develop and evolve.
Get the most from your AI Infrastructure
Ensuring your NVIDIA Clara Guardian infrastructure remains at its optimum is key to your applications continued evolution and expansion. The Scan AI Ecosystem includes a range of professional services including installation, configuration and monitoring alongside consulting for cyber security and end-to-end project management.
If necessary, we can arrange hosting of your AI infrastructure with a range of datacentre partners, based either in the UK or in a variety of Scandinavian countries to take advantage of renewable energy sources. We also provide colocation and relocation services to best suit your business and data compliance needs.
The nature of energy production in the UK often results in surplus renewable power being stranded in between the wind farms and the grid. This energy can be utilised by modular datacentres located where the surplus energy is generated, unlocking low-cost compute power and reduced costs compared to building a new permanent datacentre.
Share your NVIDIA Clara Guardian Success
Partnering with us for a case study increases brand exposure through coordinated joint-marketing with Scan and NVIDIA.
VIEW OUR CASE STUDIES >Get Started with NVIDIA Clara Guardian
What is NVIDIA Clara Parabricks?
Use the NVIDIA Clara Parabricks open-source framework to power genomic analysis and diverse bioinformatics workflows. It is a software suite for industry-standard next generation sequencing analysis on NVIDIA GPUs, consisting of everyday bioinformatics command-line tools running at blazing speeds. It is also complimented by NVIDIA RAPIDS, and NVIDIA BioNeMo.
Is NVIDIA Clara Parabricks Right for You?
To ensure NVIDIA Clara Parabricks is the right application for your datasets and challenges, Scan AI offers a free guided proof of concept (POC) trial. Our cloud-based GPU-accelerated hardware is located in UK datacentres and can be accessed securely from anywhere.
You are able to use your own data and will be supported by our expert data scientists to ensure you get the most from trying NVIDIA Clara Parabricks.
Leverage our Expertise
After your POC, our in-house team of data scientists and developers can identify and extract value from your data, speeding up application deployment.
Our team will work to provide analytical insight from your structured and unstructured data, defining a clear strategy which will be presented to your organisation, identifying quick wins, potential risks and include suggestions on how to minimise bottlenecks. Consultancy of this type is often pivotal in shaping your hardware plans too, as data driven projects always offer clearer system requirements when choosing AI infrastructure optimised for NVIDIA Clara Parabricks.
Additionally, we will train your teams to expand data science knowledge within your organisation.
Further your Knowledge
Scan AI is a certified NVIDIA Deep Learning Institute (DLI) provider. We offer a wide range of instructor-led courses to help your organisation get the most from GPU-accelerated hardware.
NVIDIA Holoscan physics-based models will scale rapidly, so the ‘Data Parallelism: How to Train Deep Learning Models on Multiple GPUs’ course is ideal to expand your understanding and prepare for your projects.
Get ready for NVIDIA Clara Parabricks
An NVIDIA AI Enterprise subscription is required to make the most of NVIDIA’s AI frameworks. Instead of assembling thousands of co-dependent libraries and APIs, AI Enterprise provides a full software stack and up to 24x7 technical support. AI Enterprise is licensed per GPU and is available on all AI systems from Scan.
Running NVIDIA Clara Parabricks software will require different hardware configurations for the development and training phases. Our health-check consultancy services verifies the suitability of your existing infrastructure to effectively run NVIDIA BioNeMo, with our experts offering advice on what upgrades to make if required.
You may have suitable existing GPU resource to effectively run NVIDIA Clara Parabricks, but installed across a variety of workstations and/or servers. Run:ai software creates a virtual GPU pool, enabling you to allocate the right amount of compute for every task; from fractions of a GPU, to multiple GPUs.
AI Infrastructure for NVIDIA Clara Parabricks
Custom-built AI workstations from Scan 3XS Systems are the perfect way to start developing with NVIDIA Clara Parabricks. Workstations can be configured with up to six NVIDIA RTX GPUs and are fine-tuned by our hardware engineers for performance, reliability and low-noise. The custom software stack includes NVIDIA Clara Parabricks, Ubuntu operating system and Docker for containerised workloads.
Custom-built AI servers from Scan 3XS Systems and NVIDIA DGX appliances, combined with PEAK:AIO software defined storage servers are the perfect way to train with NVIDIA ACE. These systems can be configured with up to eight NVIDIA GPUs and are fine-tuned by our hardware engineers for performance, reliability and low-noise. The custom software stack includes NVIDIA Clara Parabricks, operating system and management tools.
NVIDIA Clara Parabricks projects can also be developed, trained and scaled in a virtual environment using the Scan AI & Compute Cloud. This on-demand service has been developed from the ground up for intensive GPU-accelerated workloads and offers bespoke cloud instances to suit your individual projects, that can flex as your requirements develop and evolve.
Get the most from your AI Infrastructure
Ensuring your NVIDIA Clara Parabricks infrastructure remains at its optimum is key to your applications continued evolution and expansion. The Scan AI Ecosystem includes a range of professional services including installation, configuration and monitoring alongside consulting for cyber security and end-to-end project management.
If necessary, we can arrange hosting of your AI infrastructure with a range of datacentre partners, based either in the UK or in a variety of Scandinavian countries to take advantage of renewable energy sources. We also provide colocation and relocation services to best suit your business and data compliance needs.
The nature of energy production in the UK often results in surplus renewable power being stranded in between the wind farms and the grid. This energy can be utilised by modular datacentres located where the surplus energy is generated, unlocking low-cost compute power and reduced costs compared to building a new permanent datacentre.
Share your NVIDIA Clara Parabricks Success
Partnering with us for a case study increases brand exposure through coordinated joint-marketing with Scan and NVIDIA.
VIEW OUR CASE STUDIES >Get Started with NVIDIA Clara Parabricks
What is NVIDIA FLARE?
Use the NVIDIA Federated Learning Application Runtime Environment (FLARE) open-source framework to simplify the development and deployment of existing machine learning and deep learning workflows such as PyTorch, RAPIDS, NeMo or TensorFlow, to a federated paradigm. This allow the building of a secure, privacy-preserving platform for distributed multi-party collaboration. The FLARE SDK is further complemented by NVIDIA MONAI.
Is NVIDIA FLARE Right for You?
To ensure NVIDIA FLARE is the right application for your datasets and challenges, Scan AI offers a free guided proof of concept (POC) trial. Our cloud-based GPU-accelerated hardware is located in UK datacentres and can be accessed securely from anywhere.
You are able to use your own data and will be supported by our expert data scientists to ensure you get the most from trying NVIDIA FLARE.
Leverage our Expertise
After your POC, our in-house team of data scientists and developers can identify and extract value from your data, speeding up application deployment.
Our team will work to provide analytical insight from your structured and unstructured data, defining a clear strategy which will be presented to your organisation, identifying quick wins, potential risks and include suggestions on how to minimise bottlenecks. Consultancy of this type is often pivotal in shaping your hardware plans too, as data driven projects always offer clearer system requirements when choosing AI infrastructure optimised for NVIDIA FLARE.
Additionally, we will train your teams to expand data science knowledge within your organisation.
Further your Knowledge
Scan AI is a certified NVIDIA Deep Learning Institute (DLI) provider. We offer a wide range of instructor-led courses to help your organisation get the most from GPU-accelerated hardware.
NVIDIA FLARE physics-based models will scale rapidly, so the ‘Data Parallelism: How to Train Deep Learning Models on Multiple GPUs’ course is ideal to expand your understanding and prepare for your projects.
Get ready for NVIDIA FLARE
An NVIDIA AI Enterprise subscription is required to make the most of NVIDIA’s AI frameworks. Instead of assembling thousands of co-dependent libraries and APIs, AI Enterprise provides a full software stack and up to 24x7 technical support. AI Enterprise is licensed per GPU and is available on all AI systems from Scan.
Running NVIDIA FLARE software will require different hardware configurations for the development and training phases. Our health-check consultancy services verifies the suitability of your existing infrastructure to effectively run NVIDIA BioNeMo, with our experts offering advice on what upgrades to make if required.
AI Infrastructure for NVIDIA FLARE
Custom-built AI workstations from Scan 3XS Systems are the perfect way to start developing with NVIDIA FLARE. Workstations can be configured with up to six NVIDIA RTX GPUs and are fine-tuned by our hardware engineers for performance, reliability and low-noise. The custom software stack includes NVIDIA FLARE, Ubuntu operating system and Docker for containerised workloads.
Custom-built AI servers from Scan 3XS Systems and NVIDIA DGX appliances, combined with PEAK:AIO software defined storage servers are the perfect way to train with NVIDIA ACE. These systems can be configured with up to eight NVIDIA GPUs and are fine-tuned by our hardware engineers for performance, reliability and low-noise. The custom software stack includes NVIDIA FLARE, operating system and management tools.
NVIDIA FLARE projects can also be developed, trained and scaled in a virtual environment using the Scan AI & Compute Cloud. This on-demand service has been developed from the ground up for intensive GPU-accelerated workloads and offers bespoke cloud instances to suit your individual projects, that can flex as your requirements develop and evolve.
Get the most from your AI Infrastructure
Ensuring your NVIDIA FLARE infrastructure remains at its optimum is key to your applications continued evolution and expansion. The Scan AI Ecosystem includes a range of professional services including installation, configuration and monitoring alongside consulting for cyber security and end-to-end project management.
If necessary, we can arrange hosting of your AI infrastructure with a range of datacentre partners, based either in the UK or in a variety of Scandinavian countries to take advantage of renewable energy sources. We also provide colocation and relocation services to best suit your business and data compliance needs.
The nature of energy production in the UK often results in surplus renewable power being stranded in between the wind farms and the grid. This energy can be utilised by modular datacentres located where the surplus energy is generated, unlocking low-cost compute power and reduced costs compared to building a new permanent datacentre.
Share your NVIDIA FLARE Success
Partnering with us for a case study increases brand exposure through coordinated joint-marketing with Scan and NVIDIA.
VIEW OUR CASE STUDIES >Get Started with NVIDIA FLARE
What is NVIDIA Picasso?
Use the NVIDIA Picasso open source framework to train and customise state-of-the-art visual generative AI models that are both commercially safe and deployable in the cloud. Create custom visual generative AI models for your applications and services by using custom models or the included multimodal Edify architecture, using standard imagery, 3D, 360 HDRI, PBR material or video.
Is NVIDIA Picasso Right for You?
To ensure NVIDIA Picasso is the right application for your datasets and challenges, Scan AI offers a free guided proof of concept (POC) trial. Our cloud-based GPU-accelerated hardware is located in UK datacentres and can be accessed securely from anywhere.
You are able to use your own data and will be supported by our expert data scientists to ensure you get the most from trying NVIDIA Picasso.
Leverage our Expertise
After your POC, our in-house team of data scientists and developers can identify and extract value from your data, speeding up application deployment.
Our team will work to provide analytical insight from your structured and unstructured data, defining a clear strategy which will be presented to your organisation, identifying quick wins, potential risks and include suggestions on how to minimise bottlenecks. Consultancy of this type is often pivotal in shaping your hardware plans too, as data driven projects always offer clearer system requirements when choosing AI infrastructure optimised for NVIDIA Picasso.
Additionally, we will train your teams to expand data science knowledge within your organisation.
Further your Knowledge
Scan AI is a certified NVIDIA Deep Learning Institute (DLI) provider. We offer a wide range of instructor-led courses to help your organisation get the most from GPU-accelerated hardware.
NVIDIA Picasso models will scale rapidly, so the ‘Data Parallelism: How to Train Deep Learning Models on Multiple GPUs’ course is ideal to expand your understanding and prepare for your projects.
AI Infrastructure for NVIDIA Picasso
NVIDIA Picasso projects can only be run on NVIDIA DGX Cloud infrastructure, designed for training and inferencing complex AI models it delivers outstanding performance in visual LLMs and generative AI. The Scan Cloud team can help provision DGX Cloud instances for your organisation.
Share your NVIDIA Picasso Success
Partnering with us for a case study increases brand exposure through coordinated joint-marketing with Scan and NVIDIA.
VIEW OUR CASE STUDIES >Get Started with NVIDIA Picasso
What is NVIDIA Maxine?
Use the NVIDIA Maxine open source framework to deploy AI features that enhance audio, video and add augmented reality effects in real time. Maxine’s state-of-the-art models create high-quality effects that can be achieved with standard microphone and camera equipment. Maxine can be deployed on-premise, in the cloud, or at the edge, and it provides a complete audio and video enhancement pipeline of multiple low-latency effects chained together, complimenting those delivered by NVIDIA Riva and NVIDIA ACE.
Is NVIDIA Maxine Right for You?
To ensure NVIDIA Maxine is the right application for your datasets and challenges, Scan AI offers a free guided proof of concept (POC) trial. Our cloud-based GPU-accelerated hardware is located in UK datacentres and can be accessed securely from anywhere.
You are able to use your own data and will be supported by our expert data scientists to ensure you get the most from trying NVIDIA Maxine.
Leverage our Expertise
After your POC, our in-house team of data scientists and developers can identify and extract value from your data, speeding up application deployment.
Our team will work to provide analytical insight from your structured and unstructured data, defining a clear strategy which will be presented to your organisation, identifying quick wins, potential risks and include suggestions on how to minimise bottlenecks. Consultancy of this type is often pivotal in shaping your hardware plans too, as data driven projects always offer clearer system requirements when choosing AI infrastructure optimised for NVIDIA Maxine.
Additionally, we will train your teams to expand data science knowledge within your organisation.
Further your Knowledge
Scan AI is a certified NVIDIA Deep Learning Institute (DLI) provider. We offer a wide range of instructor-led courses to help your organisation get the most from GPU-accelerated hardware.
NVIDIA Maxine physics-based models will scale rapidly, so the ‘Data Parallelism: How to Train Deep Learning Models on Multiple GPUs’ course is ideal to expand your understanding and prepare for your projects.
Get ready for NVIDIA Maxine
An NVIDIA AI Enterprise subscription is required to make the most of NVIDIA’s AI frameworks. Instead of assembling thousands of co-dependent libraries and APIs, AI Enterprise provides a full software stack and up to 24x7 technical support. AI Enterprise is licensed per GPU and is available on all AI systems from Scan.
Running NVIDIA Maxine software will require different hardware configurations for the development and training phases. Our health-check consultancy services verifies the suitability of your existing infrastructure to effectively run NVIDIA BioNeMo, with our experts offering advice on what upgrades to make if required.
AI Infrastructure for NVIDIA Maxine
Custom-built AI workstations from Scan 3XS Systems are the perfect way to start developing with NVIDIA Maxine. Workstations can be configured with up to six NVIDIA RTX GPUs and are fine-tuned by our hardware engineers for performance, reliability and low-noise. The custom software stack includes NVIDIA Maxine, Ubuntu operating system and Docker for containerised workloads.
Custom-built AI servers from Scan 3XS Systems and NVIDIA DGX appliances, combined with PEAK:AIO software defined storage servers are the perfect way to train with NVIDIA ACE. These systems can be configured with up to eight NVIDIA GPUs and are fine-tuned by our hardware engineers for performance, reliability and low-noise. The custom software stack includes NVIDIA Maxine, operating system and management tools.
NVIDIA Maxine projects can also be developed, trained and scaled in a virtual environment using the Scan AI & Compute Cloud. This on-demand service has been developed from the ground up for intensive GPU-accelerated workloads and offers bespoke cloud instances to suit your individual projects, that can flex as your requirements develop and evolve.
Get the most from your AI Infrastructure
Ensuring your NVIDIA Maxine infrastructure remains at its optimum is key to your applications continued evolution and expansion. The Scan AI Ecosystem includes a range of professional services including installation, configuration and monitoring alongside consulting for cyber security and end-to-end project management.
If necessary, we can arrange hosting of your AI infrastructure with a range of datacentre partners, based either in the UK or in a variety of Scandinavian countries to take advantage of renewable energy sources. We also provide colocation and relocation services to best suit your business and data compliance needs.
The nature of energy production in the UK often results in surplus renewable power being stranded in between the wind farms and the grid. This energy can be utilised by modular datacentres located where the surplus energy is generated, unlocking low-cost compute power and reduced costs compared to building a new permanent datacentre.
Share your NVIDIA Maxine Success
Partnering with us for a case study increases brand exposure through coordinated joint-marketing with Scan and NVIDIA.
VIEW OUR CASE STUDIES >Get Started with NVIDIA Maxine
What is NVIDIA Metropolis?
Use the NVIDIA Metropolis open source framework to combines visual data and AI to improve operational efficiency and safety across a range of industries. It helps make sense of data created by trillions of sensors for some of the world’s most valuable physical transactions. These include frictionless retail, streamlined inventory management, traffic engineering in smart cities, optical inspection on factory floors, patient care in healthcare facilities and more. Enterprises can take advantage of Metropolis developer tools and ecosystem to create, deploy, and scale AI and IoT applications from the edge to the cloud. Metropolis is supported by NVIDIA DeepStream, NVIDIA TAO Toolkit and available in NVIDIA Omnverse.
Is NVIDIA Metropolis Right for You?
To ensure NVIDIA Metropolis is the right application for your datasets and challenges, Scan AI offers a free guided proof of concept (POC) trial. Our cloud-based GPU-accelerated hardware is located in UK datacentres and can be accessed securely from anywhere.
You are able to use your own data and will be supported by our expert data scientists to ensure you get the most from trying NVIDIA Metropolis.
Leverage our Expertise
After your POC, our in-house team of data scientists and developers can identify and extract value from your data, speeding up application deployment.
Our team will work to provide analytical insight from your structured and unstructured data, defining a clear strategy which will be presented to your organisation, identifying quick wins, potential risks and include suggestions on how to minimise bottlenecks. Consultancy of this type is often pivotal in shaping your hardware plans too, as data driven projects always offer clearer system requirements when choosing AI infrastructure optimised for NVIDIA Metropolis.
Additionally, we will train your teams to expand data science knowledge within your organisation.
Further your Knowledge
Scan AI is a certified NVIDIA Deep Learning Institute (DLI) provider. We offer a wide range of instructor-led courses to help your organisation get the most from GPU-accelerated hardware.
NVIDIA Metropolis physics-based models will scale rapidly, so the ‘Data Parallelism: How to Train Deep Learning Models on Multiple GPUs’ course is ideal to expand your understanding and prepare for your projects.
Get ready for NVIDIA Metropolis
An NVIDIA AI Enterprise subscription is required to make the most of NVIDIA’s AI frameworks. Instead of assembling thousands of co-dependent libraries and APIs, AI Enterprise provides a full software stack and up to 24x7 technical support. AI Enterprise is licensed per GPU and is available on all AI systems from Scan.
Running NVIDIA Metropolis software will require different hardware configurations for the development and training phases. Our health-check consultancy services verifies the suitability of your existing infrastructure to effectively run NVIDIA BioNeMo, with our experts offering advice on what upgrades to make if required.
You may have suitable existing GPU resource to effectively run NVIDIA Metropolis, but installed across a variety of workstations and/or servers. Run:ai software creates a virtual GPU pool, enabling you to allocate the right amount of compute for every task; from fractions of a GPU, to multiple GPUs.
AI Infrastructure for NVIDIA Metropolis
Custom-built AI workstations from Scan 3XS Systems are the perfect way to start developing with NVIDIA Metropolis. Workstations can be configured with up to six NVIDIA RTX GPUs and are fine-tuned by our hardware engineers for performance, reliability and low-noise. The custom software stack includes NVIDIA Metropolis, Ubuntu operating system and Docker for containerised workloads.
Custom-built AI servers from Scan 3XS Systems and NVIDIA DGX appliances, combined with PEAK:AIO software defined storage servers are the perfect way to train with NVIDIA ACE. These systems can be configured with up to eight NVIDIA GPUs and are fine-tuned by our hardware engineers for performance, reliability and low-noise. The custom software stack includes NVIDIA Metropolis, operating system and management tools.
NVIDIA Metropolis projects can also be developed, trained and scaled in a virtual environment using the Scan AI & Compute Cloud. This on-demand service has been developed from the ground up for intensive GPU-accelerated workloads and offers bespoke cloud instances to suit your individual projects, that can flex as your requirements develop and evolve.
Get the most from your AI Infrastructure
Ensuring your NVIDIA Metropolis infrastructure remains at its optimum is key to your applications continued evolution and expansion. The Scan AI Ecosystem includes a range of professional services including installation, configuration and monitoring alongside consulting for cyber security and end-to-end project management.
If necessary, we can arrange hosting of your AI infrastructure with a range of datacentre partners, based either in the UK or in a variety of Scandinavian countries to take advantage of renewable energy sources. We also provide colocation and relocation services to best suit your business and data compliance needs.
The nature of energy production in the UK often results in surplus renewable power being stranded in between the wind farms and the grid. This energy can be utilised by modular datacentres located where the surplus energy is generated, unlocking low-cost compute power and reduced costs compared to building a new permanent datacentre.
Share your NVIDIA Metropolis Success
Partnering with us for a case study increases brand exposure through coordinated joint-marketing with Scan and NVIDIA.
VIEW OUR CASE STUDIES >Get Started with NVIDIA Metropolis
What is NVIDIA Aerial?
Use the NVIDIA Aerial open source framework to build high-performance, software-defined, GPU-accelerated, cloud-native 5G networks that deliver high performance to customers and improved TCO to service providers. Aerial is a suite of accelerated computing platforms, software and services for designing, simulating, and operating wireless networks. Aerial contains hardened RAN software libraries for telcos, cloud service providers (CSPs), and enterprises building commercial 5G networks. Academic and industry researchers can access Aerial on cloud or on-premises setups for advanced wireless and AI and machine learning (ML) research for 6G.
Is NVIDIA Aerial Right for You?
To ensure NVIDIA Aerial is the right application for your datasets and challenges, Scan AI offers a free guided proof of concept (POC) trial. Our cloud-based GPU-accelerated hardware is located in UK datacentres and can be accessed securely from anywhere.
You are able to use your own data and will be supported by our expert data scientists to ensure you get the most from trying NVIDIA Aerial.
Leverage our Expertise
After your POC, our in-house team of data scientists and developers can identify and extract value from your data, speeding up application deployment.
Our team will work to provide analytical insight from your structured and unstructured data, defining a clear strategy which will be presented to your organisation, identifying quick wins, potential risks and include suggestions on how to minimise bottlenecks. Consultancy of this type is often pivotal in shaping your hardware plans too, as data driven projects always offer clearer system requirements when choosing AI infrastructure optimised for NVIDIA Aerial.
Additionally, we will train your teams to expand data science knowledge within your organisation.
Further your Knowledge
Scan AI is a certified NVIDIA Deep Learning Institute (DLI) provider. We offer a wide range of instructor-led courses to help your organisation get the most from GPU-accelerated hardware.
NVIDIA Aerial physics-based models will scale rapidly, so the ‘Data Parallelism: How to Train Deep Learning Models on Multiple GPUs’ course is ideal to expand your understanding and prepare for your projects.
Get ready for NVIDIA Aerial
An NVIDIA AI Enterprise subscription is required to make the most of NVIDIA’s AI frameworks. Instead of assembling thousands of co-dependent libraries and APIs, AI Enterprise provides a full software stack and up to 24x7 technical support. AI Enterprise is licensed per GPU and is available on all AI systems from Scan.
Running NVIDIA Aerial software will require different hardware configurations for the development and training phases. Our health-check consultancy services verifies the suitability of your existing infrastructure to effectively run NVIDIA BioNeMo, with our experts offering advice on what upgrades to make if required.
AI Infrastructure for NVIDIA Aerial
Custom-built AI workstations from Scan 3XS Systems are the perfect way to start developing with NVIDIA Aerial. Workstations can be configured with up to six NVIDIA RTX GPUs and are fine-tuned by our hardware engineers for performance, reliability and low-noise. The custom software stack includes NVIDIA Aerial, Ubuntu operating system and Docker for containerised workloads.
Custom-built AI servers from Scan 3XS Systems and NVIDIA DGX appliances, combined with PEAK:AIO software defined storage servers are the perfect way to train with NVIDIA ACE. These systems can be configured with up to eight NVIDIA GPUs and are fine-tuned by our hardware engineers for performance, reliability and low-noise. The custom software stack includes NVIDIA Aerial, operating system and management tools.
NVIDIA Aerial projects can also be developed, trained and scaled in a virtual environment using the Scan AI & Compute Cloud. This on-demand service has been developed from the ground up for intensive GPU-accelerated workloads and offers bespoke cloud instances to suit your individual projects, that can flex as your requirements develop and evolve.
Get the most from your AI Infrastructure
Ensuring your NVIDIA Aerial infrastructure remains at its optimum is key to your applications continued evolution and expansion. The Scan AI Ecosystem includes a range of professional services including installation, configuration and monitoring alongside consulting for cyber security and end-to-end project management.
If necessary, we can arrange hosting of your AI infrastructure with a range of datacentre partners, based either in the UK or in a variety of Scandinavian countries to take advantage of renewable energy sources. We also provide colocation and relocation services to best suit your business and data compliance needs.
The nature of energy production in the UK often results in surplus renewable power being stranded in between the wind farms and the grid. This energy can be utilised by modular datacentres located where the surplus energy is generated, unlocking low-cost compute power and reduced costs compared to building a new permanent datacentre.
Share your NVIDIA Aerial Success
Partnering with us for a case study increases brand exposure through coordinated joint-marketing with Scan and NVIDIA.
VIEW OUR CASE STUDIES >Get Started with NVIDIA Aerial
What is NVIDIA Rivermax?
Use the NVIDIA Rivermax open source framework to deliver IP-based solutions for any media and data streaming applications. Rivermax leverages NVIDIA ConnectX and BlueField DPU hardware-streaming acceleration technology to enable direct data transfers to and from GPUs, delivering superior throughput and latency with minimal CPU utilisation for streaming workloads. It is the only fully-virtualised streaming solution that complies with the stringent timing and traffic flow requirements of the SMPTE ST 2110-21 specification. Rivermax enables innovation for a wide range of applications in Media and Entertainment, Broadcast, Healthcare and Smart Cities.
Is NVIDIA Rivermax Right for You?
To ensure NVIDIA Rivermax is the right application for your datasets and challenges, Scan AI offers a free guided proof of concept (POC) trial. Our cloud-based GPU-accelerated hardware is located in UK datacentres and can be accessed securely from anywhere.
You are able to use your own data and will be supported by our expert data scientists to ensure you get the most from trying NVIDIA Rivermax.
Leverage our Expertise
After your POC, our in-house team of data scientists and developers can identify and extract value from your data, speeding up application deployment.
Our team will work to provide analytical insight from your structured and unstructured data, defining a clear strategy which will be presented to your organisation, identifying quick wins, potential risks and include suggestions on how to minimise bottlenecks. Consultancy of this type is often pivotal in shaping your hardware plans too, as data driven projects always offer clearer system requirements when choosing AI infrastructure optimised for NVIDIA Rivermax.
Additionally, we will train your teams to expand data science knowledge within your organisation.
Further your Knowledge
Scan AI is a certified NVIDIA Deep Learning Institute (DLI) provider. We offer a wide range of instructor-led courses to help your organisation get the most from GPU-accelerated hardware.
NVIDIA Rivermax physics-based models will scale rapidly, so the ‘Data Parallelism: How to Train Deep Learning Models on Multiple GPUs’ course is ideal to expand your understanding and prepare for your projects.
Get ready for NVIDIA Rivermax
An NVIDIA AI Enterprise subscription is required to make the most of NVIDIA’s AI frameworks. Instead of assembling thousands of co-dependent libraries and APIs, AI Enterprise provides a full software stack and up to 24x7 technical support. AI Enterprise is licensed per GPU and is available on all AI systems from Scan.
Running NVIDIA Rivermax software will require different hardware configurations for the development and training phases. Our health-check consultancy services verifies the suitability of your existing infrastructure to effectively run NVIDIA Rivermax, with our experts offering advice on what upgrades to make if required.
AI Infrastructure for NVIDIA Rivermax
Custom-built AI workstations from Scan 3XS Systems are the perfect way to start developing with NVIDIA Rivermax. Workstations can be configured with up to six NVIDIA RTX GPUs and are fine-tuned by our hardware engineers for performance, reliability and low-noise. The custom software stack includes NVIDIA Rivermax, Ubuntu operating system and Docker for containerised workloads.
Custom-built AI servers from Scan 3XS Systems and NVIDIA DGX appliances, combined with PEAK:AIO software defined storage servers are the perfect way to get the most from NVIDIA Rivermax. These systems can be configured with up to eight GPUs and either NVIDIA ConnectX Smart NICs and BlueField DPUs alongside a custom software stack including NVIDIA Rivermax, operating system and management tools.
NVIDIA Rivermax projects can also be developed, trained and scaled in a virtual environment using the Scan AI & Compute Cloud. This on-demand service has been developed from the ground up for intensive GPU-accelerated workloads and offers bespoke cloud instances to suit your individual projects, that can flex as your requirements develop and evolve.
Get the most from your AI Infrastructure
Ensuring your NVIDIA Rivermax infrastructure remains at its optimum is key to your applications continued evolution and expansion. The Scan AI Ecosystem includes a range of professional services including installation, configuration and monitoring alongside consulting for cyber security and end-to-end project management.
If necessary, we can arrange hosting of your AI infrastructure with a range of datacentre partners, based either in the UK or in a variety of Scandinavian countries to take advantage of renewable energy sources. We also provide colocation and relocation services to best suit your business and data compliance needs.
The nature of energy production in the UK often results in surplus renewable power being stranded in between the wind farms and the grid. This energy can be utilised by modular datacentres located where the surplus energy is generated, unlocking low-cost compute power and reduced costs compared to building a new permanent datacentre.
Share your NVIDIA Rivermax Success
Partnering with us for a case study increases brand exposure through coordinated joint-marketing with Scan and NVIDIA.
VIEW OUR CASE STUDIES >Get Started with NVIDIA Rivermax
What is NVIDIA DeepStream?
Use the NVIDIA DeepStream open source framework to power streaming analytics for AI-based multi-sensor processing, video, audio and image understanding. The DeepStream SDK enables developers to create stream processing pipelines that incorporate neural networks and other complex processing tasks such as tracking, video encoding / decoding and video rendering. DeepStream is an integral part of NVIDIA Metropolis, as its pipelines enable real-time analytics on video, image, and sensor data. DeepStream can be further enhanced using NVIDIA Rivermax optimised networking SDK.
Is NVIDIA DeepStream Right for You?
To ensure NVIDIA DeepStream is the right application for your datasets and challenges, Scan AI offers a free guided proof of concept (POC) trial. Our cloud-based GPU-accelerated hardware is located in UK datacentres and can be accessed securely from anywhere.
You are able to use your own data and will be supported by our expert data scientists to ensure you get the most from trying NVIDIA DeepStream.
Leverage our Expertise
After your POC, our in-house team of data scientists and developers can identify and extract value from your data, speeding up application deployment.
Our team will work to provide analytical insight from your structured and unstructured data, defining a clear strategy which will be presented to your organisation, identifying quick wins, potential risks and include suggestions on how to minimise bottlenecks. Consultancy of this type is often pivotal in shaping your hardware plans too, as data driven projects always offer clearer system requirements when choosing AI infrastructure optimised for NVIDIA DeepStream.
Additionally, we will train your teams to expand data science knowledge within your organisation.
Further your Knowledge
Scan AI is a certified NVIDIA Deep Learning Institute (DLI) provider. We offer a wide range of instructor-led courses to help your organisation get the most from GPU-accelerated hardware.
NVIDIA DeepStream physics-based models will scale rapidly, so the ‘Data Parallelism: How to Train Deep Learning Models on Multiple GPUs’ course is ideal to expand your understanding and prepare for your projects.
Get ready for NVIDIA DeepStream
An NVIDIA AI Enterprise subscription is required to make the most of NVIDIA’s AI frameworks. Instead of assembling thousands of co-dependent libraries and APIs, AI Enterprise provides a full software stack and up to 24x7 technical support. AI Enterprise is licensed per GPU and is available on all AI systems from Scan.
Running NVIDIA DeepStream software will require different hardware configurations for the development and training phases. Our health-check consultancy services verifies the suitability of your existing infrastructure to effectively run NVIDIA DeepStream, with our experts offering advice on what upgrades to make if required.
You may have suitable existing GPU resource to effectively run NVIDIA DeepStream, but installed across a variety of workstations and/or servers. Run:ai software creates a virtual GPU pool, enabling you to allocate the right amount of compute for every task; from fractions of a GPU, to multiple GPUs.
AI Infrastructure for NVIDIA DeepStream
Custom-built AI workstations from Scan 3XS Systems are the perfect way to start developing with NVIDIA DeepStream. Workstations can be configured with up to six NVIDIA RTX GPUs and are fine-tuned by our hardware engineers for performance, reliability and low-noise. The custom software stack includes NVIDIA DeepStream, Ubuntu operating system and Docker for containerised workloads.
Custom-built AI servers from Scan 3XS Systems and NVIDIA DGX appliances, combined with PEAK:AIO software defined storage servers are the perfect way to train with NVIDIA DeepStream. These systems can be configured with up to eight NVIDIA GPUs and are fine-tuned by our hardware engineers for performance, reliability and low-noise. The custom software stack includes NVIDIA Aerial, operating system and management tools.
NVIDIA DeepStream projects can also be developed, trained and scaled in a virtual environment using the Scan AI & Compute Cloud. This on-demand service has been developed from the ground up for intensive GPU-accelerated workloads and offers bespoke cloud instances to suit your individual projects, that can flex as your requirements develop and evolve.
Get the most from your AI Infrastructure
Ensuring your NVIDIA DeepStream infrastructure remains at its optimum is key to your applications continued evolution and expansion. The Scan AI Ecosystem includes a range of professional services including installation, configuration and monitoring alongside consulting for cyber security and end-to-end project management.
If necessary, we can arrange hosting of your AI infrastructure with a range of datacentre partners, based either in the UK or in a variety of Scandinavian countries to take advantage of renewable energy sources. We also provide colocation and relocation services to best suit your business and data compliance needs.
The nature of energy production in the UK often results in surplus renewable power being stranded in between the wind farms and the grid. This energy can be utilised by modular datacentres located where the surplus energy is generated, unlocking low-cost compute power and reduced costs compared to building a new permanent datacentre.
Share your NVIDIA DeepStream Success
Partnering with us for a case study increases brand exposure through coordinated joint-marketing with Scan and NVIDIA.
VIEW OUR CASE STUDIES >Get Started with NVIDIA DeepStream
What is NVIDIA Riva?
Use the NVIDIA Riva open source framework to build and deploy fully customisable multilingual speech and translation AI applications. Riva includes automatic speech recognition (ASR), text-to-speech (TTS), neural machine translation (NMT) and is deployable in the cloud, in the datacentre or at the edge. With Riva, organisations can add speech and translation interfaces with large language models (LLMs) and retrieval-augmented generation (RAG) to transform chatbots into engaging and expressive multilingual assistants, and avatars when used with NVIDIA ACE.
Is NVIDIA Riva Right for You?
To ensure NVIDIA Riva is the right application for your datasets and challenges, Scan AI offers a free guided proof of concept (POC) trial. Our cloud-based GPU-accelerated hardware is located in UK datacentres and can be accessed securely from anywhere.
You are able to use your own data and will be supported by our expert data scientists to ensure you get the most from trying NVIDIA Riva.
Leverage our Expertise
After your POC, our in-house team of data scientists and developers can identify and extract value from your data, speeding up application deployment.
Our team will work to provide analytical insight from your structured and unstructured data, defining a clear strategy which will be presented to your organisation, identifying quick wins, potential risks and include suggestions on how to minimise bottlenecks. Consultancy of this type is often pivotal in shaping your hardware plans too, as data driven projects always offer clearer system requirements when choosing AI infrastructure optimised for NVIDIA Riva.
Additionally, we will train your teams to expand data science knowledge within your organisation.
Further your Knowledge
Scan AI is a certified NVIDIA Deep Learning Institute (DLI) provider. We offer a wide range of instructor-led courses to help your organisation get the most from GPU-accelerated hardware.
NVIDIA Riva physics-based models will scale rapidly, so the ‘Data Parallelism: How to Train Deep Learning Models on Multiple GPUs’ course is ideal to expand your understanding and prepare for your projects.
Get ready for NVIDIA Riva
An NVIDIA AI Enterprise subscription is required to make the most of NVIDIA’s AI frameworks. Instead of assembling thousands of co-dependent libraries and APIs, AI Enterprise provides a full software stack and up to 24x7 technical support. AI Enterprise is licensed per GPU and is available on all AI systems from Scan.
Running NVIDIA Riva software will require different hardware configurations for the development and training phases. Our health-check consultancy services verifies the suitability of your existing infrastructure to effectively run NVIDIA DeepStream, with our experts offering advice on what upgrades to make if required.
AI Infrastructure for NVIDIA Riva
Custom-built AI workstations from Scan 3XS Systems are the perfect way to start developing with NVIDIA Riva. Workstations can be configured with up to six NVIDIA RTX GPUs and are fine-tuned by our hardware engineers for performance, reliability and low-noise. The custom software stack includes NVIDIA Riva, Ubuntu operating system and Docker for containerised workloads.
Custom-built AI servers from Scan 3XS Systems and NVIDIA DGX appliances, combined with PEAK:AIO software defined storage servers are the perfect way to train with NVIDIA Riva. These systems can be configured with up to eight NVIDIA GPUs and are fine-tuned by our hardware engineers for performance, reliability and low-noise. The custom software stack includes NVIDIA Aerial, operating system and management tools.
NVIDIA Riva projects can also be developed, trained and scaled in a virtual environment using the Scan AI & Compute Cloud. This on-demand service has been developed from the ground up for intensive GPU-accelerated workloads and offers bespoke cloud instances to suit your individual projects, that can flex as your requirements develop and evolve.
Get the most from your AI Infrastructure
Ensuring your NVIDIA Riva infrastructure remains at its optimum is key to your applications continued evolution and expansion. The Scan AI Ecosystem includes a range of professional services including installation, configuration and monitoring alongside consulting for cyber security and end-to-end project management.
If necessary, we can arrange hosting of your AI infrastructure with a range of datacentre partners, based either in the UK or in a variety of Scandinavian countries to take advantage of renewable energy sources. We also provide colocation and relocation services to best suit your business and data compliance needs.
The nature of energy production in the UK often results in surplus renewable power being stranded in between the wind farms and the grid. This energy can be utilised by modular datacentres located where the surplus energy is generated, unlocking low-cost compute power and reduced costs compared to building a new permanent datacentre.
Share your NVIDIA Riva Success
Partnering with us for a case study increases brand exposure through coordinated joint-marketing with Scan and NVIDIA.
VIEW OUR CASE STUDIES >