AI projects development and scale rapidly and can consume huge amounts of GPU-accelerated resource alongside speciliast storage and networking overheads. To address these challenges, the Scan AI Ecosystem includes managed hosting options. We’ve partnered with a number of secure datacentres to deliver tailor-made hardware hosting environments delivering high performance and unlimited scalability, while providing security and peace of mind. Organisations maintain control over their own systems but without the day-to-day admin or complex racking, power and cooling concerns, associated with on-premise AI infrastructure.
Benefits of Managed Hosting
Scan AI managed hosting services, delivered in conjunction with our datacentre partners offer numerous benefits when compared to on-premise hardware solutions.
Expert Support
Unlike other remote GPU-accelerated services, Scan AI hosting services are supported by a full team of expert consultants including data scientists, Linux engineers and hardware architects.
Cost Efficiency
Hosting removes the need to plan and build costly server rooms within your premises plus the additional expense of employing and training technical staff to support and maintain them.
Simple Scalability
AI projects always grow, but expansion is simple as any additional resource requirements are planned and scoped by the datacentre team removing risks and costs.
Secure Access
Login via a variety of secured connection options with physical segregation, advanced firewalls and zero resource sharing.
Guaranteed Uptime
Many of our partners are certified Tier 1 datacentres delivering multiple paths for power and cooling, focusing on fault tolerance and greater than 99.9% uptime.
Sustainable Energy
Some of our datacentre partners provide 100% renewable energy sources for power and cooling such as hydro-electric or geothermal energy.
Approved Datacentres
Several of our partners have the NVIDIA DGX-ready accreditation meaning they have been optimised to provide the best in AI supercomputing.
Consultancy Services
Scan AI provides help and advice with all frameworks, algorithms and software, including assistance in interpretation of your deep learning and AI results.
Free POC Trial
The Scan AI team, in conjunction with many of our datacentre providers, offer a proof of concept trial so you have peace of mind prior to choosing your hosting partner.
Our Managed Hosting Partners
We have partnered with a number of datacentres, including Tier 1-3 options, UK and European-based locations and modular datacentres.
Interxion operates numerous datacentres across London and in other major cities around the UK, to provide cost-effective low-latency Tier 1 facilities and interconnect services.
LEARN MORE >Verne DGX-ready datacentres harness the sustainable powers of Icelandic hydroelectric and geothermal energy to provide cost-sensitive Tier 3 facilities for high density infrastructures.
LEARN MORE >Kao Data datacentre facilities are located east and west of London and feature DGX-ready Tier 3 architectures, powered by 100% renewable energy and aimed solely at HPC and AI customers.
LEARN MORE >Cyxtera operate a Tier 1 NVIDIA certified, DGX-ready datacentre close to London, focused on delivering cost-effective services for colocation and other customisable, flexible solutions.
LEARN MORE >Unitas Global offers datacentre connectivity solutions including Unitas Reach - designed to deploy and manage cloud infrastructure and networking solutions for enterprise clients, all over the world.
LEARN MORE >Green Mountain datacentres are some of the world’s greenest using 100% renewable hydropower to operate and cool award-winning, high efficiency Tier 3 facilities, located deep inside Norwegian mountains.
LEARN MORE >iomart operate multiple Tier 1 datacentres across the UK offering the ability to cost-effectively colocate any IT infrastructure and delivering simple connectivity, security and scalability.
LEARN MORE >The Goonhilly datacentre is part of the Earth Station infrastructure located in Cornwall and offers cost-effective Tier 3 hosting solutions aimed at space-related HPC and AI research.
LEARN MORE >Energy production in the UK often results in surplus renewable power being stranded in-between wind farms and the grid. Scan AI can provision modular datacentres in these locations to take advantage of lower energy costs.
LEARN MORE >Start Hosting your Infrastructure
Scan is an NVIDIA Elite Partner and many of our hosting partners are certified NVIDIA DGX-ready datacentres, so our offerings have been designed with GPU-accelerated systems in mind. On top of this Scan will fully manage your AI hardware relocation to any of our datacentre partners including shipment and forwarding, import paperwork and customs clearance, VAT registration and reclaim, installation and deployment. This end-to-end service ensures your organisation has complete peace of mind when undertaking a large infrastructure relocation. Having provided this service numerous times for our clients we have developed a slick process that we believe cannot be matched.
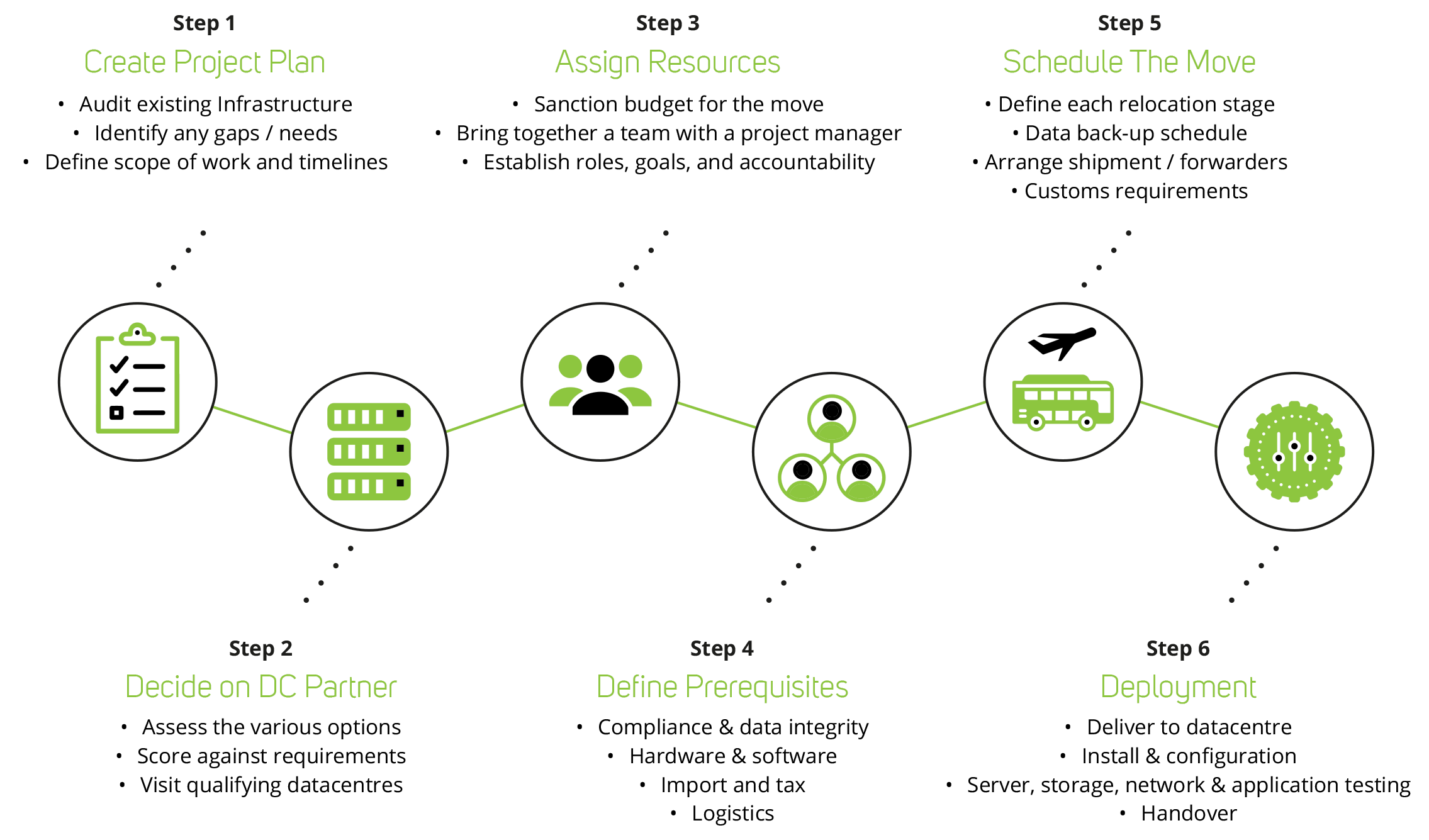
Get in touch with our AI experts on the details below to discuss any requirement or challenges you may have.
Enquire now