Get in touch with our AI team.
Run:ai Cluster Management
AI Infrastructure Management for Innovators
Run:ai's platform revolutionises AI and machine learning operations by addressing key infrastructure challenges through dynamic resource allocation, comprehensive AI lifecycle support, and strategic resource management. By pooling resources across environments and utilising advanced orchestration and accelerators, Run:ai significantly enhances GPU efficiency and workload capacity.
Its policy engine and open architecture foster strategic alignment with business objectives, enabling seamless integration with external tools and systems. This results in significant increases in GPU availability, workloads, and GPU utilisation, all with zero manual resource intervention, accelerating innovation and providing a scalable, agile, and cost-effective solution for enterprises.
AI Infrastructure Management
AI Infrastructure Management represents a transformative approach to managing and optimising AI resources and operations within your enterprise. It is an ecosystem designed to overcome the inherent challenges in traditional AI infrastructure by being dynamic, strategic, and integrally aligned with business objectives. The Run:ai infrastructure management platform revolutionises the way enterprises manage and optimise their AI and machine learning operations. This platform is specifically designed to address the unique challenges of AI infrastructure, enhancing efficiency, scalability, and flexibility.
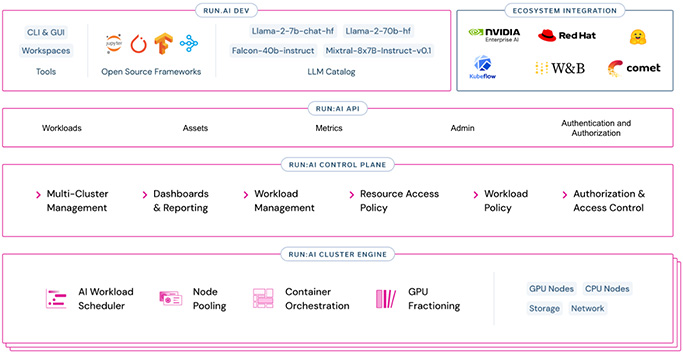
Promote practitioner productivity with the Run:ai GUI. Run:ai makes it simple for a practitioner to access compute and run workloads without being a technical expert. Workspaces and templates were built with end users in mind.