AI Training Appliance by NVIDIA
The fifth-generation DGX datacentre AI appliances are built around the Hopper architecture and the flagship H200 accelerators, providing unprecedented training and inferencing performance in a single system.
The DGX H200 include 400Gb/s Connect-X7 Smart NICs and Bluefield DPUs for connecting to external storage, supported by the NVIDIA Base Command management suite and the NVIDIA AI Enterprise software stack, backed by specialist technical advice from NVIDIA DGXperts.
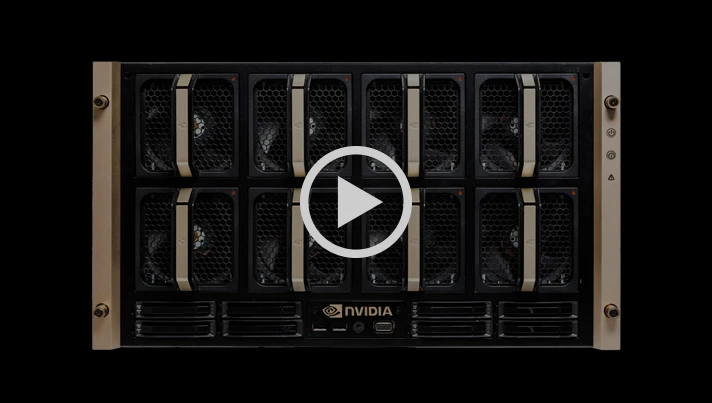
Accelerated AI with NVIDIA Hopper GPUs
The DGX H200 features eight SXM5 Hopper Tensor Core GPUs, each with 141GB of memory, providing a minimum of 6x more performance than previous generation DGX appliances. The H200’s larger and faster memory accelerates generative AI and LLMs, with better energy efficiency and lower total cost of ownership. Based on the Hopper architecture they feature 4th gen NVLink and 3rd gen NVSwitch technology to deliver maximum performance for all workloads. Additionally 2nd gen Multiple Instance GPU (MIG) offers the ability to virtualise each GPU into seven discreet instances.
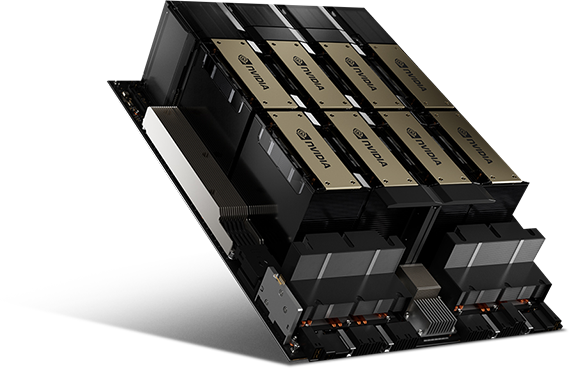
Specifications | |||
---|---|---|---|
ARCHITECTURE | Hopper | ||
GPU | H200 | ||
CUDA CORES | 16,896 | ||
TENSOR CORES | 528 4th gen | ||
MEMORY | 141GB HBM3e | ||
ECC MEMORY | ✓ | ||
MEMORY CONTROLLER | 5,120-bit | ||
NVLINK SPEED | 900GB/sec |
AI-Ready Software Stack
NVIDIA Base Command powers the DGX systems, enabling organisations to leverage the best of NVIDIA software innovation. Enterprises can unleash the full potential of their DGX infrastructure with a proven platform that includes enterprise-grade orchestration and cluster management, libraries that accelerate compute, storage and network infrastructure, and an operating system optimised for AI workloads. This is further enhanced by NVIDIA AI Enterprise.
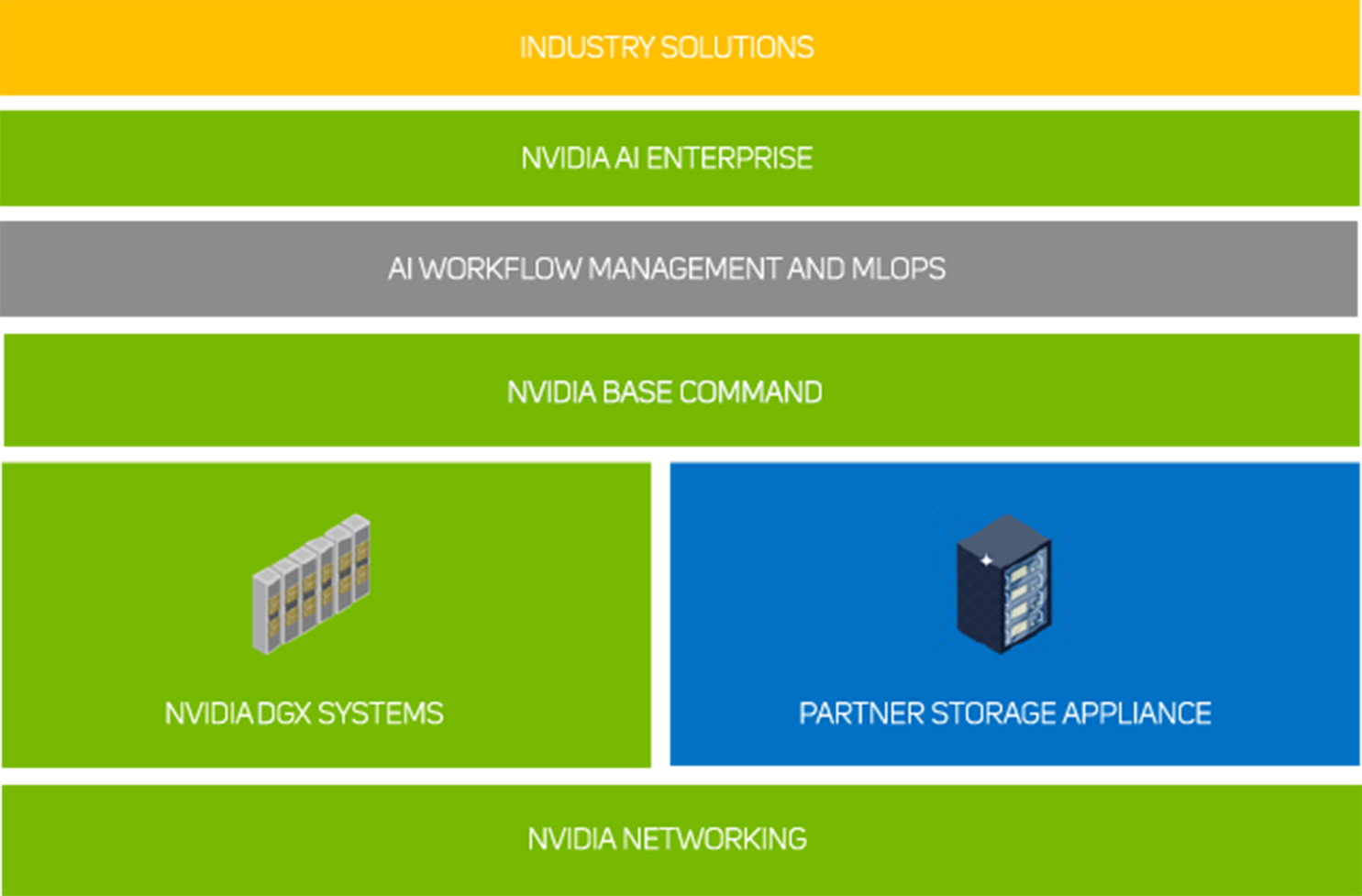
NVIDIA AI Enterprise
NVIDIA AI Enterprise unlocks access to a wide range of frameworks that accelerate the development and deployment of AI projects. Leveraging pre-configured frameworks removes many of the manual tasks and complexity associated with software development, enabling you to deploy your AI models faster as each framework is tried, tested and optimised for NVIDIA GPUs. The less time spent developing, the greater the ROI on your AI hardware and data science investments.
Rather than trying to assemble thousands of co-dependent libraries and APIs from different authors when building your own AI applications, NVIDIA AI Enterprise removes this pain point by providing the full AI software stack including applications such as healthcare, computer vision, speech and generative AI.
Enterprise-grade support is provided, 9x5 with a 4-hour SLA with direct access to NVIDIA’s AI experts, minimising risk and downtime, while maximising system efficiency and productivity. A three-year NVIDIA AI Enterprise license is included with 3XS AI workstations with A800 GPUs as standard. You can also purchase one, three- and five-year licenses with other GPUs.
Workload Management
Run:ai software allows intelligent resource management and consumption so that users can easily access GPU fractions, multiple GPUs or clusters of servers for workloads of every size and stage of the AI lifecycle. This ensures that all available compute can be utilised and GPUs never have to sit idle. Run:ai’s scheduler is a simple plug-in for Kubernetes clusters and adds high-performance orchestration to your containerised AI workloads.
FIND OUT MORE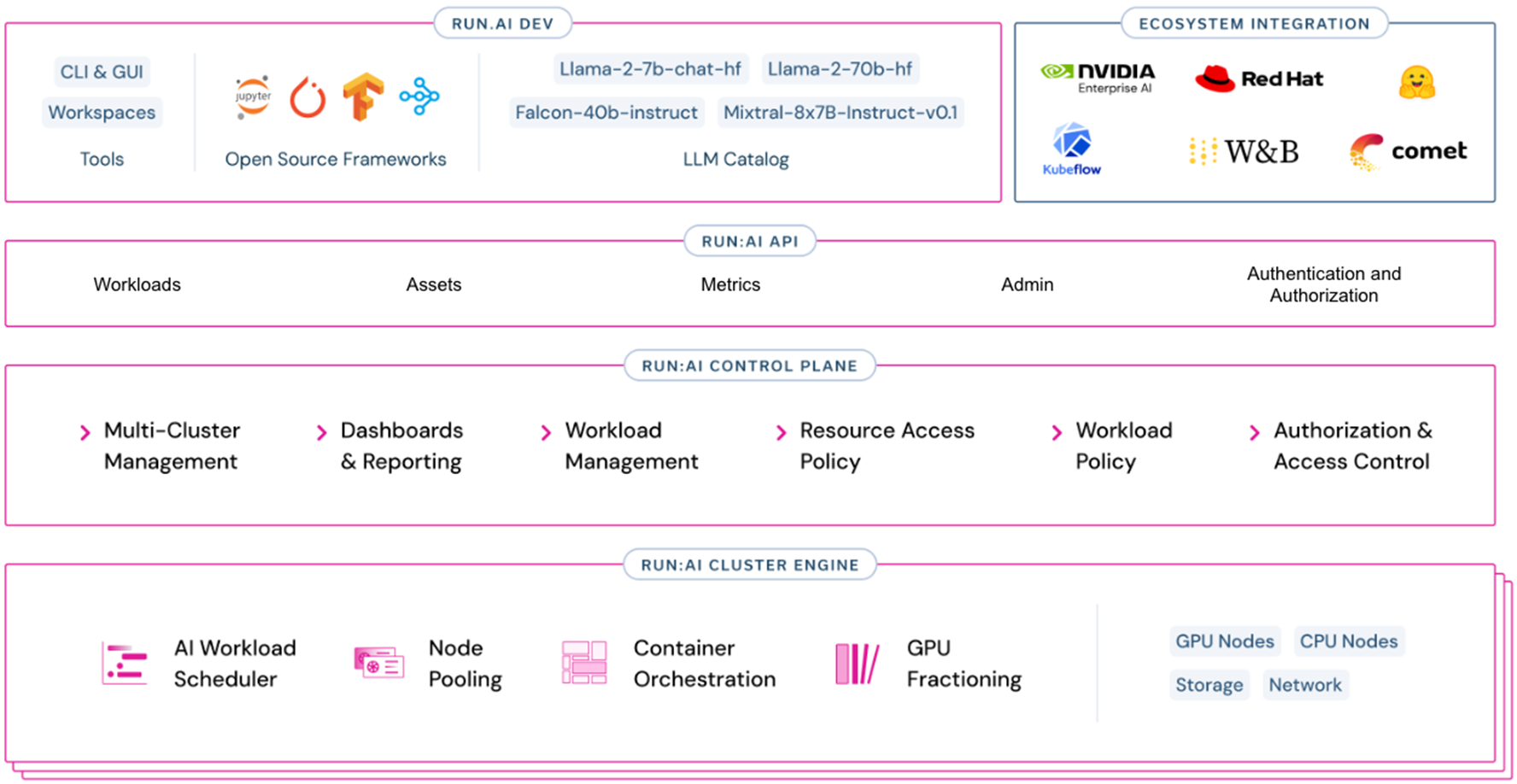
AI Optimised Storage
AI Optimised storage appliances ensure that your NVIDIA DGX systems are being utilised as much as possible and always working at maximum efficiency. Scan AI offers software-defined storage appliances powered by PEAK:AIO and further options from leading brands such as Dell-EMC, NetApp and DDN to ensure we have an AI optimised storage solution that is right for you.
FIND OUT MORE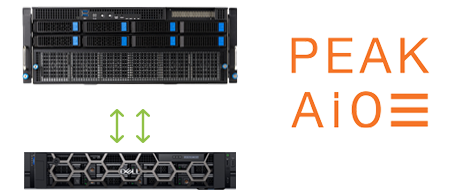
Managed Hosting Solutions
AI projects scale rapidly and can consume huge amounts of GPU-accelerated resource alongside significant storage and networking overheads. To address these challenges, the Scan AI Ecosystem includes managed hosting options. We’ve partnered with a number of secure datacentre partners to deliver tailor-made hardware hosting environments delivering high performance and unlimited scalability, while providing security and peace of mind. Organisations maintain control over their own systems but without the day-to-day admin or complex racking, power and cooling concerns, associated with on-premise infrastructure.
FIND OUT MORE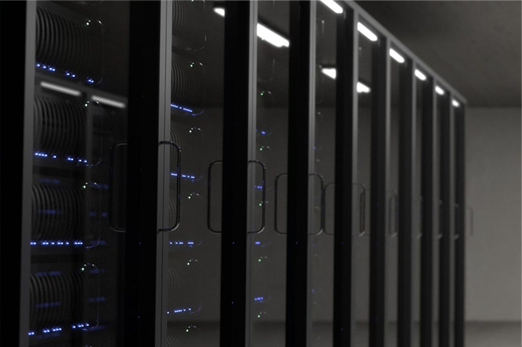
NVIDIA BasePOD & SuperPOD
DGX BasePOD and SuperPOD are NVIDIA reference architectures based around a specific infrastructure kit list that Scan can configure and deploy into your organisation to deliver AI at scale.
PODs start as small as 20 DGX nodes, scaling all the way to 140 nodes, managed by a comprehensive software stack to form a complete cluster.
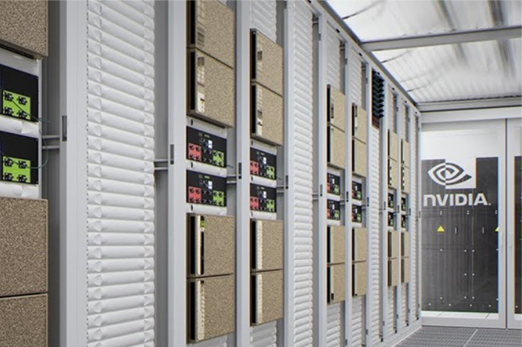
Start your DGX Journey
The NVIDIA DGX H200 AI appliance is available with three- and five-year support contract, extendable at a later period. There are also comprehensive media retention packages available for more data-sensitive projects.
GPU:8x NVIDIA H200 141GB - 1,128GB total
CPU: 2x Intel Xeon Platinum 8480C – 112 cores / 224 threads total
RAM: 2TB ECC Reg DDR5
System Drives: 2x 1.92TB NVMe SSDs
Storage Drives: 8x 3.84TB NVMe SSDs
Networking: 8x 400Gb/s NVIDIA ConnectX-7 InfiniBand/Ethernet and 2x 400Gb/s NVIDIA Bluefield DPUs InfiniBand/Ethernet
Power: 10.2kW
Form Factor: 8U
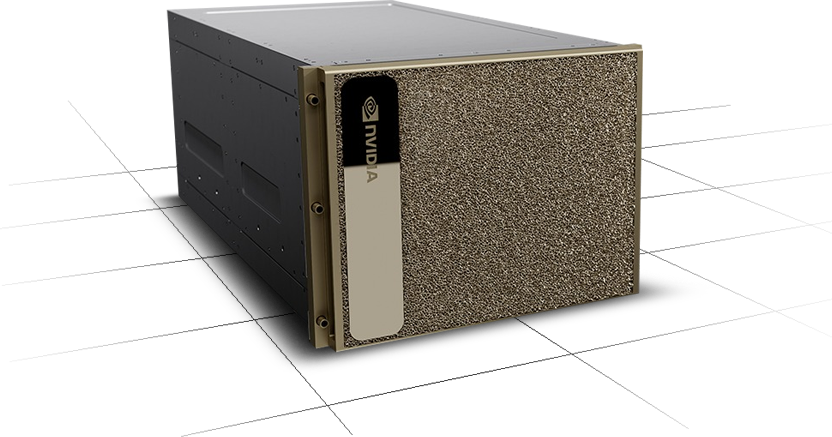