AI Training & Inferencing Servers by 3XS Systems
3XS custom AI servers for training and inferencing are based on NVIDIA-certified EGX designs. Powered by NVIDIA professional PCIe GPUs, they provide data scientists and researchers with a flexible, cost-effective platform for scaling-out AI models.
Designed for datacentre environments, 3XS AI servers are fine-tuned by our hardware engineers and data scientists for maximum performance and reliability. We provide a custom software stack that means you spend more time on your model training and less time configuring drivers, libraries and APIs.
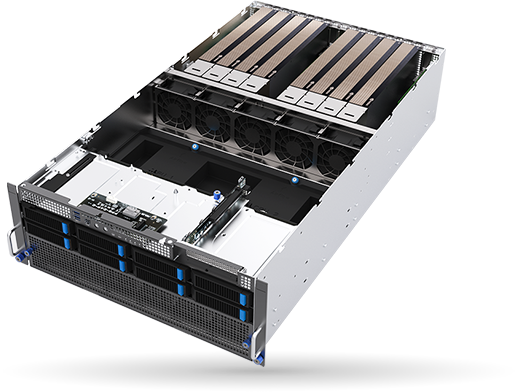
Accelerated AI with NVIDIA GPUs
The choice and number of GPUs has the most impact on how long AI models take to train and inference. 3XS custom AI servers support up to eight NVIDIA GPUs for the ultimate in performance. Explore the range of NVIDIA GPUs in more detail below
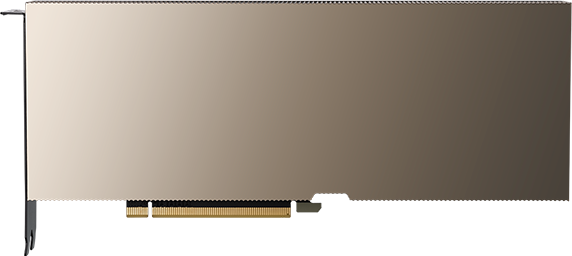
RTX PRO Blackwell Server | H200 NVL | L40S | RTX 6000 ADA | L4 | |
---|---|---|---|---|---|
ARCHITECTURE | Blackwell | Hopper | Ada Lovelace | Ada Lovelace | Ada Lovelace |
BUS | PCIe 5 | PCIe 5 | PCIe 4 | PCIe 4 | PCIe 4 |
GPU | GB202 | H200 | AD102 | AD102 | AD104 |
CUDA CORES | 24,064 | 16,896 | 18,176 | 18,176 | 7,680 |
TENSOR CORES | 752 5th gen | 528 4th gen | 568 4th gen | 568 4th gen | 240 4th gen |
MEMORY | 96GB GDDR7 | 141GB HBM3 | 48GB GDDR6 | 48GB GDDR6 | 24GB GDDR6 |
ECC MEMORY | ✔ | ✔ | ✔ | ✔ | ✔ |
MEMORY CONTROLLER | 512-bit | 5,120-bit | 384-bit | 384-bit | 192-bit |
NVLINK SPEED | ✖ | 900GB/sec | ✖ | ✖ | ✖ |
TDP | 600W | 600W | 350W | 300W | 72W |
AI-Ready Software Stack
3XS AI servers are provided with a custom software stack to speed up your AI training. This includes the latest Ubuntu operating system, Docker for creating, sharing and running individual containers on a single node and TensorFlow, a popular framework for building and deploying AI applications.
Powered by NVIDIA GPUs, 3XS AI servers are the perfect tool for unlocking the comprehensive catalogue of optimised software tools for AI and HPC provided in the NVIDIA GPU Cloud (NGC).
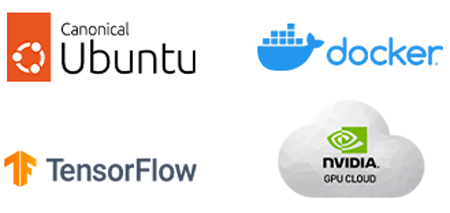
NVIDIA AI Enterprise
NVIDIA AI Enterprise unlocks access to a wide range of frameworks that accelerate the development and deployment of AI projects. Leveraging pre-configured frameworks removes many of the manual tasks and complexity associated with software development, enabling you to deploy your AI models faster as each framework is tried, tested and optimised for NVIDIA GPUs. The less time spent developing, the greater the ROI on your AI hardware and data science investments.
Rather than trying to assemble thousands of co-dependent libraries and APIs from different authors when building your own AI applications, NVIDIA AI Enterprise removes this pain point by providing the full AI software stack including applications such as healthcare, computer vision, speech and generative AI.
Enterprise-grade support is provided, 9x5 with a 4-hour SLA with direct access to NVIDIA’s AI experts, minimising risk and downtime, while maximising system efficiency and productivity. A three-year NVIDIA AI Enterprise license is included with 3XS AI workstations with A800 GPUs as standard. You can also purchase one, three- and five-year licenses with other GPUs.
Workload Management
Run:ai software allows intelligent resource management and consumption so that users can easily access GPU fractions, multiple GPUs or clusters of servers for workloads of every size and stage of the AI lifecycle. This ensures that all available compute can be utilised and GPUs never have to sit idle. Run:ai’s scheduler is a simple plug-in for Kubernetes clusters and adds high-performance orchestration to your containerised AI workloads.
FIND OUT MORE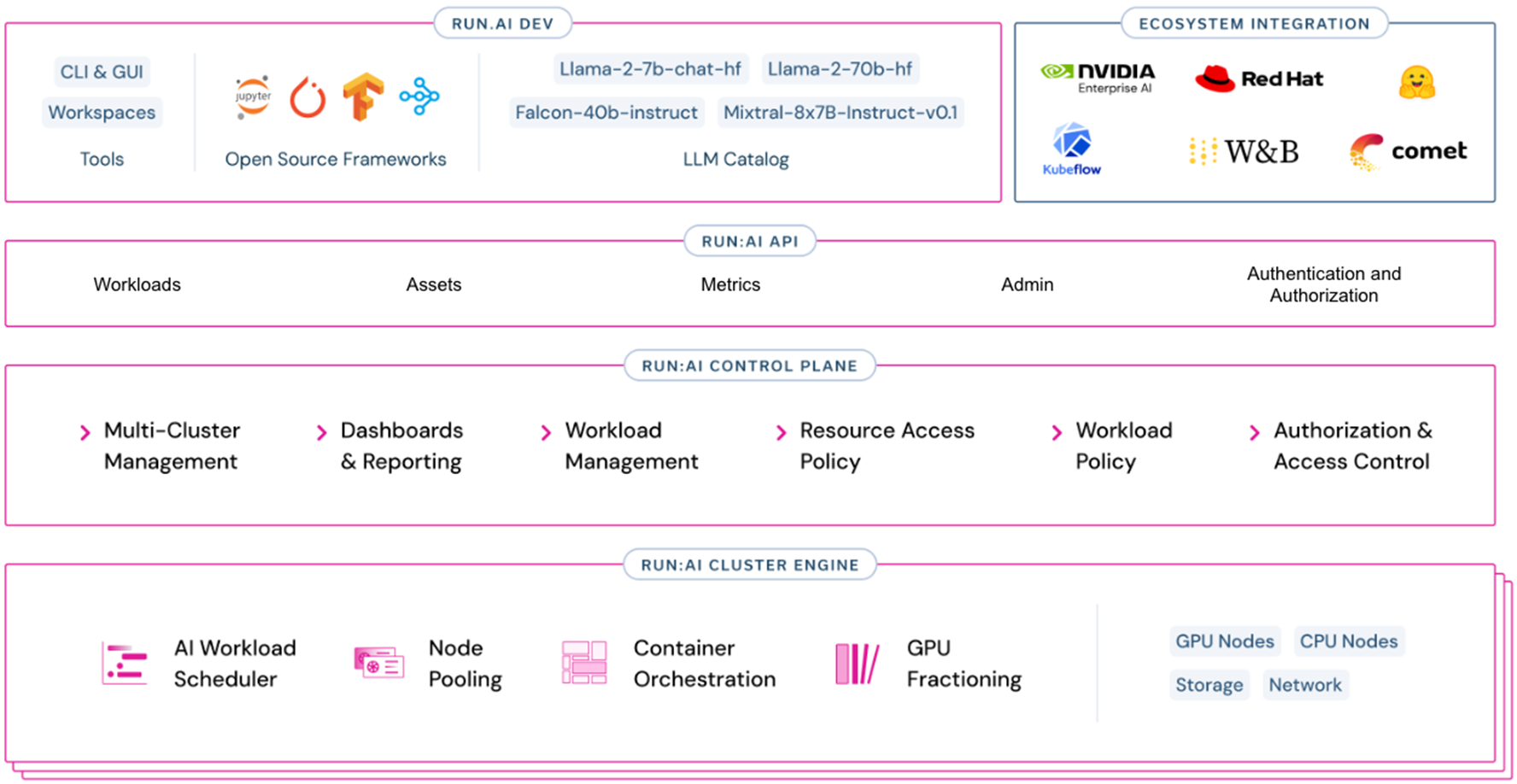
AI Optimised Storage
AI Optimised storage appliances ensure that your NVIDIA DGX systems are being utilised as much as possible and always working at maximum efficiency. Scan AI offers software-defined storage appliances powered by PEAK:AIO and further options from leading brands such as Dell-EMC, NetApp and DDN to ensure we have an AI optimised storage solution that is right for you.
FIND OUT MORE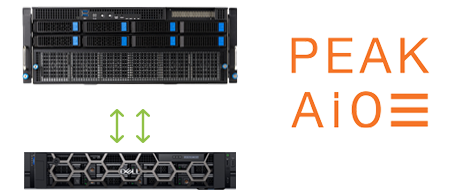
Managed Hosting Solutions
AI projects scale rapidly and can consume huge amounts of GPU-accelerated resource alongside significant storage and networking overheads. To address these challenges, the Scan AI Ecosystem includes managed hosting options. We’ve partnered with a number of secure datacentre partners to deliver tailor-made hardware hosting environments delivering high performance and unlimited scalability, while providing security and peace of mind. Organisations maintain control over their own systems but without the day-to-day admin or complex racking, power and cooling concerns, associated with on-premise infrastructure.
FIND OUT MORE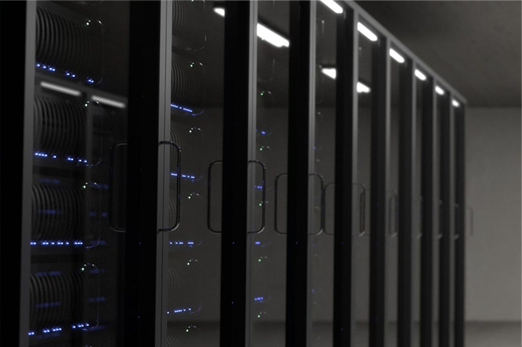
Select your 3XS EGX Custom AI Server
3XS AI servers are available in a range of base configurations that can be customised to your requirements.
![]() |
![]() |
![]() |
![]() |
|
GPUs | Up to 4x NVIDIA PCIe cards | Up to 4x NVIDIA PCIe cards | Up to 8x NVIDIA PCIe cards | Up to 8x NVIDIA PCIe cards |
CPUs | 1x AMD EPYC up to 128-cores | 2x Intel Xeon up to 64-cores | 2x AMD EPYC up to 128-cores | 2x Intel Xeon up to 64-cores |
Cooling | Air / Water | Air / Water | Air / Water | Air / Water |
RAM | Up to 1TB | Up to 2TB | Up to 4TB | Up to 2TB |
Storage | 1x M.2, 2x NVMe, 6x SATA | 1x M.2, 2x NVMe, 6x SATA | 1x M.2, 2x NVMe, 6x SATA | 1x M.2, 2x NVMe, 6x SATA |
Networking | NVIDIA Connect-X NICs or Bluefield DPUs up to 800Gb/s | NVIDIA Connect-X NICs or Bluefield DPUs up to 800Gb/s | NVIDIA Connect-X NICs or Bluefield DPUs up to 800Gb/s | NVIDIA Connect-X NICs or Bluefield DPUs up to 800Gb/s |
Form Factor | 2U | 2U | 4U | 4U |
If you’re still unsure of the best solution for your organisation, contact our team to discuss your projects or requirements and to arrange a free GUIDED PROOF OF CONCEPT TRIAL Alternatively, you can train AI models on any device via virtualised NVIDIA GPUs hosted on our SCAN CLOUD PLATFORM