Our Aim
To provide you with an overview on New And existing technologies, hopefully helping you understand the changes in the technology. Together with the overviews we hope to bring topical issues to light from a series of independent reviewers saving you the time And hassle of fact finding over the web.
We will over time provide you with quality content which you can browse and subscribe to at your leisure.
TekSpek 's

NVIDIA DGX-2 Supercomputer
Date issued:
The increase in processing speed for computers has opened up new avenues of research and provided deeper insights into solutions for complex problems. Such power has been harnessed to accelerate research into medicine, better predict weather patterns, perform hugely complex calculations for the oil and gas industry, and a whole host more.
One area that has especially benefitted from a fantastic amount of horsepower is machine learning, where through a process of training and inference a machine is able to gain what humans consider to be artificial intelligence (AI).
Machines develop this AI by running complex algorithms that are designed to recognise patterns in workloads. For example, by examining millions upon millions of images and classifying each based on preset parameters that correlate to the right result - this is how grass ought to look, this is how a cat ought to move, etc. - the deep-learning computer learns which patterns lead to the right result. This is the training part. The second part, inference, refers to the ability of the computer to understand that it is looking at the correct pattern.
Examples of successful machine learning and inference can be found in practically all fields. Sound, audio, images, and video are but a few, and it has hugely practical benefits in medicine, where deep-learning computers can be taught to identify potentially cancerous moles through studying millions of examples. Recent studies have shown that adequately-trained machines are better able to distinguish between cancerous and non-cancerous moles than healthcare professionals when looking at high-resolution images.
It is accepted that machine learning is going to change the way we process information, but the challenge remains one of building a hardware and software infrastructure capable of handling today's modern AI workloads. This is where NVIDIA, a world leader in computer graphics, is at the forefront of machine learning and AI through its DGX deep-learning systems.
Sifting through millions of training inputs takes considerable computational time, of course, and the parallel-processing ability of GPUs is key into processing them as fast as possible. This is why a computer outfitted with a number of specific-purpose, cutting-edge GPUs is so good at the training portion of deep learning.
The NVIDIA DGX-2 is the world's most powerful deep learning system for the most complex AI challenges, according to NVIDIA. In fact, one DGX-2 has the same training ability - which is the time-consuming part - as 300 servers filled with dual Intel Xeon Gold CPUs.
Anyone interested in proper deep learning ought to understand just what makes the DGX-2 so good at deep learning and training, so let's take a look at what's under the hood.
The solution: NVIDIA DGX-2
System Specifications | |
---|---|
GPUs | 16x NVIDIA® Tesla V100 |
GPU Memory | 512GB total |
Performance | 2petaFLOPS |
NVIDIA CUDA® Cores | 81920 |
NVIDIA Tensor Cores | 10240 |
NVSwitches | 12 |
Maximum Power Usage | 10kW |
CPU | Dual Intel Xeon Platinum 8168,2.7GHz, 24-cores |
System Memory | 1.5TB |
Network | 8x 100Gb/sec Infiniband/100GigE Dual 10/25Gb/sec Ethernet |
Storage | OS: 2X 960GB NVME SSDs Internal Storage: 30TB (8X 3.84TB) NVME SSDs |
Software | Unbuntu Linux OS See Software stack for details |
System Weight | 340 lbs (154.2 kgs) |
System Dimensions | Height: 17.3 in (440.0 mm) Width: 19.0 in (482.3mm) Length: 31.3 in (795.4 mm) - No Front Bezel 32.8 in (834.0 mm) - With Front Bezel |
Operating Temperature range | 5°C to 35°C (41°F to 95°F) |
The key component of the DGX-2 deep-learning system is the 16 fully-connected Nvidia Tesla V100 'Volta' 32GB GPUs, each composed of 5,120 general-processing CUDA and 640 learning-optimised Tensor cores. The GPU's design is different from any previous generation because it amalgamates specific Tensor hardware for the very first time.
This massive horsepower gives a single DGX-2 box up to two petaflops of compute performance which is able to accelerate the newest deep-learning model types that were previously untrainable on a single system. With ground-breaking GPU scale, you can train models 4x bigger from one machine.
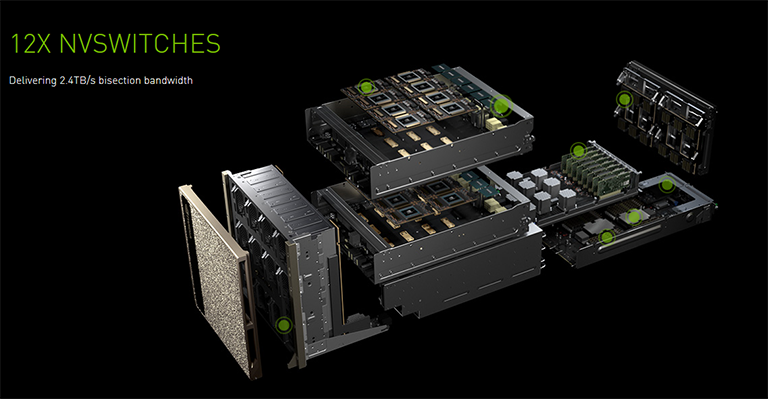
Sheer compute output is mighty impressive, as one would expect from a world leader in graphics, and is necessary to handle increasingly complex training workloads. What's equally impressive is that NVIDIA has built, from scratch, a network fabric - the ability to communicate with other GPUs, in other words - that delivers 2.4TB/s of bisection bandwidth for a 24x increase over prior generations. Known as NVSwitch, such inter-GPU bandwidth is necessary to speed-up computation on very large datasets.
Having ultra-impressive hardware specifications is one thing, but one needs excellent supporting software to maximise its potential. Nvidia has a complete DGX-2 software stack for this very purpose, comprising a specialist graphics driver, and in-house deep-learning user software known as DIGITS. One top of this run all of the major machine-learning frameworks such as Caffe, TensorFlow, mxnet, theano, and torch.
Absolute computational performance is everything with respect to the training phase of machine learning. The previous DGX-1 system, released last year, set a new bar on what was possible from a single computer. Today's DGX-2 takes performance into a whole new realm, as evidenced by the graphic below.
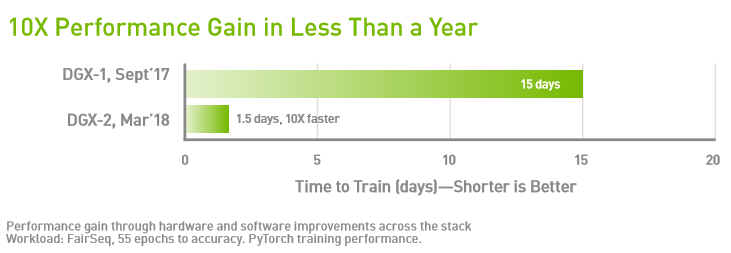
A complex AI workload that previously took 15 days to train now takes just 1.5 - a 10x improvement in less than a year. Running the same workload on a high-performance consumer computer would take many months, if not years, and as alluded to above, the DGX-2 computes and trains at the same level as 300 servers!
The point is that the more complete the training input, the more accurate the end result, and having the ability to run far more complex training datasets is an obvious boon for machine-learning advocates.
Any company or educational/research establishment serious about developing their machine-learning program needs to invest in the right tool for the job. As of September 2018, the undisputed leader in the field is NVIDIA, whose DGX-2 'supercomputer' serves as a training monster.
It is accepted that machine/deep learning and increasingly-intelligent AI is here to stay. The accuracy of the end result is contingent on having incredible processing power at the front end. And there is nothing more powerful than the DGX-2 right now.
Scan Computers an official NVIDIA solution provider, to find out more about our AI solutions you can visit our deep learning section.