FDL Europe 2022 - Live Twin: Aerosols
Understanding how aerosols and other emissions from extreme fires affect weather and climate
Published 12 JAN 2023
Frontier Development Lab (FDL) is a public-private partnership with ESA in Europe and NASA in the USA. FDL works with commercial partners to apply AI technologies to space science, to push the frontiers of research and develop new tools to help solve some of the biggest challenges that humanity faces. These range from the effects of climate change to predicting space weather, from improving disaster response, to identifying meteorites that could hold the key to the history of our universe.
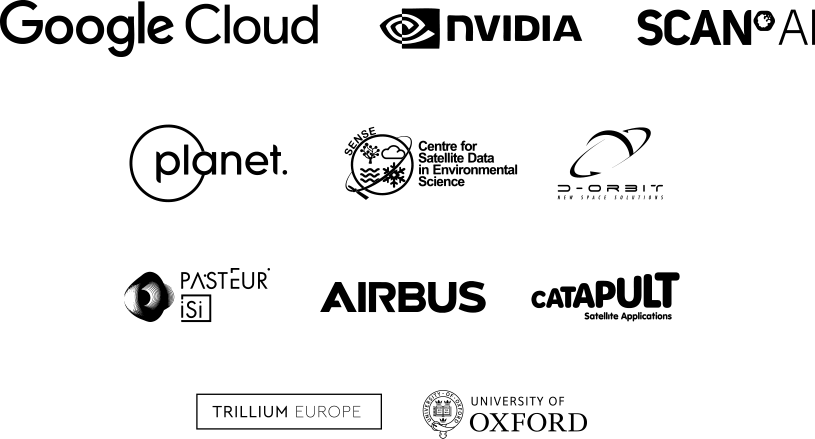
FDL Europe 2022 was a research sprint hosted by the University of Oxford that took place over a period of eight weeks in order to promote rapid learning and research outcomes in a collaborative atmosphere, pairing machine learning expertise with AI technologies and space science. The interdisciplinary teams address tightly defined problems and the format encourages rapid iteration and prototyping to create meaningful outputs to the space program and humanity.
Live Twin - Aerosols
Project Background
More than 17 million people have been affected through major wildfire events over the last 30 years. In addition, the degradation of air quality due to the creation of aerosols and ozone from fires resulted in between 260,000 and 600,000 premature deaths each year. The risk that wildfires pose to people and the environment is increasing due to climate change - it is estimated that 4% of global vegetation is destroyed by wildfires every year. By the end of the century, the frequency of wildfires, compared to a 2000 - 2010 reference period, is predicted to increase by a factor of 1.36 to 1.57, as shown below, over the next 70 - 80 years. With the number of extreme wildfires increasing even further due to events including higher air temperatures, lower relative humidity, dry lightning and strong winds. These will all ultimately result in drier, hotter and longer fire seasons.
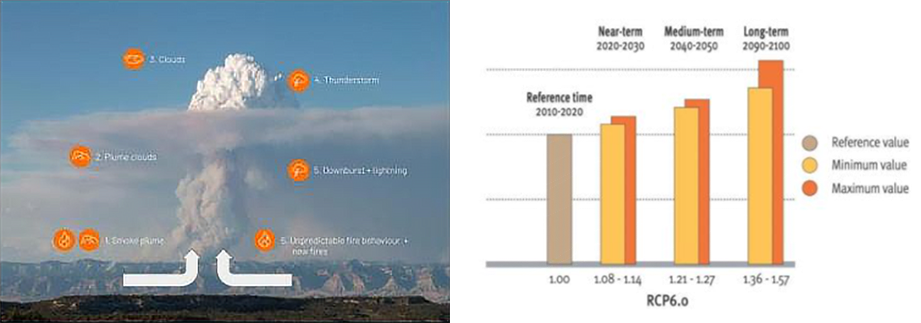
Pyrocumulonimbus or pyroCbs are storm clouds produced by particularly large and intense wildfires that can produce storm clouds. These storm clouds create their own weather fronts which can make wildfire behaviour unpredictable through strong winds and ignite new fires through lightning. PyroCbs also convect wildfire aerosols into the stratosphere, where they remain for several months. These events, which can be on the scale of a volcanic eruption, have important impacts on the Earth’s climate. PyroCbs could also hinder the recovery of the ozone layer. Despite the risk posed by pyroCbs, the conditions leading to their occurrence and evolution are still poorly understood. In this FDL challenge, the Aerosols team applied machine learning to previously collected data with the aim of monitoring, forecasting, and understanding the drivers behind pyroCb events.
Project Approach
The study of pyroCb-producing wildfires was carried out by the development of three distinct components - firstly, a master database derived from existing sources including the Australian pyroCb Registry, the Cooperative Institute for Meteorological Studies (CIMSS) pyroCb Blog and the GlobFire database. These were combined with pre-labelled geo-stationary satellite images from the GOES 16, GOES 17 and Himawari-8 satellites over the geographical areas shown in the diagram below. This data was then overlaid with meteorological and fuel data, associated with wildfire events, collected from ERA5 (the ECMWF or European Centre for Medium Range Weather Forecasts Re-Analysis in its 5th generation), including vegetation cover and type, vertical wind velocities, relative humidity and boundary layer height measurements from the atmosphere between 100m and 2000m above ground surface.
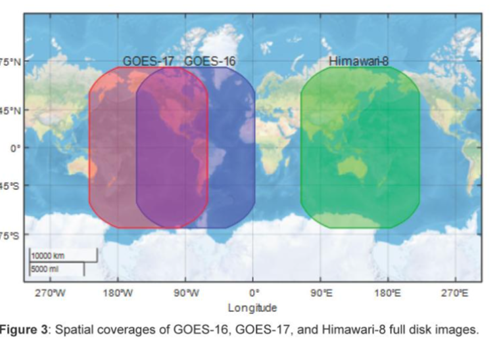
Secondly, a convolutional neural network (CNN) that takes cropped geostationary satellite images from the master database and attempts to create a random forest forecasting model algorithm that discriminates between pyroCb clouds and non-pyroCb clouds, as illustrated below. The CNN consisted of six convolutional layers and two fully connected layers, also shown below, to deliver a trained model to attempt to predict where pyroCb events may be caused and how they will behave.
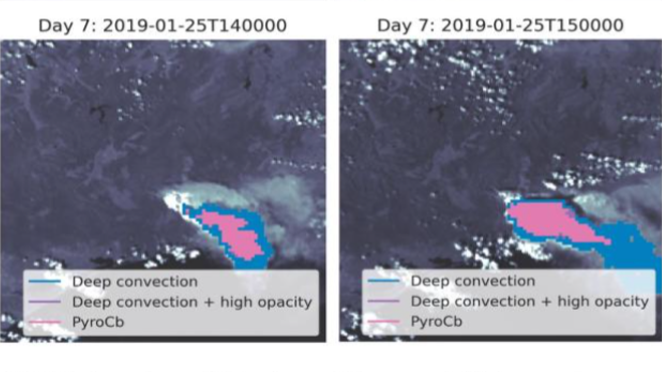
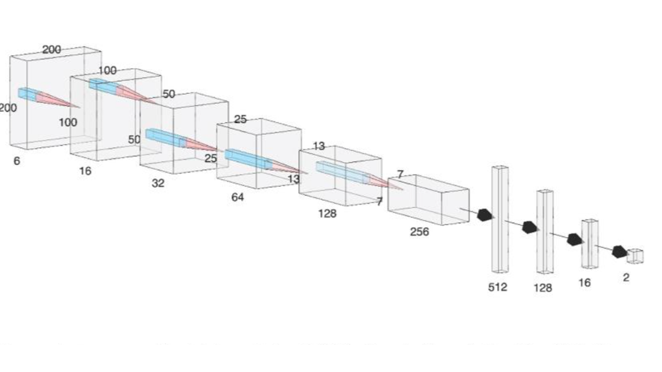
Finally, a framework set of tools was established to understand the causes and characteristics of pyroCb occurrences to give context to the algorithm’s predictions. These master database, forecasting model and framework segments were then combined into a pipeline which the team named Pyrocast. The flowchart of how these three elements work together is illustrated in the diagram below.
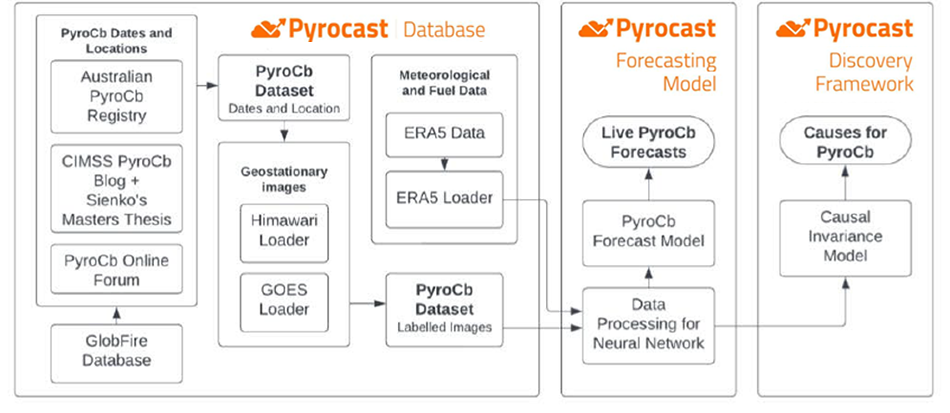
Project Results
Pyrocast is the first generation of an end-to-end pipeline to monitor, forecast and understand the drivers behind pyroCb clouds caused by extreme wildfires. The Pyrocast database provides the most comprehensive pyroCb dates with labelled geostationary satellite images and associated data for 111 individual wildfire events. The Pyrocast forecasting model has demonstrated an ability to predict behaviour over a six-hour horizon with the Pyrocast Discovery Framework showing that the most important predictors of pyroCb events were shown to be boundary height layer, followed by convective available potential energy (CAPE) and surface-level zonal wind velocity.
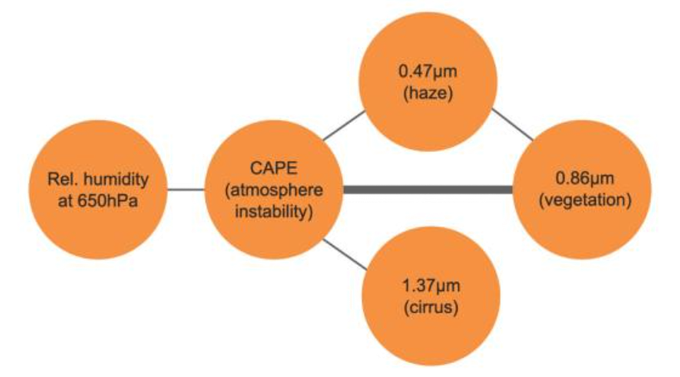
The Aerosols FDL project team concluded that although useful in its first iteration, the Pyrocast pipeline can be further refined by including more data from future pyroCb events and by reducing the geostationary satellite sampling data window to every 10 minutes. They further proposed more pertaining on non-wildfire geostationary images to further enhance the model’s predictability. You can learn more about this case study by reading the FDL 2022 Research Booklet, where a poster and full Technical Memorandum can also be downloaded.
The Scan Partnership
NVIDIA is a key supporter of the Frontier Development Lab and the FDL Europe 2022 event, and Scan AI was asked to act as a technology partner of NVIDIA to provide access to multiple DGX appliances in order to facilitate much of the machine learning and deep learning development and training required. The FDL Aerosols team used Google Cloud Platform (GCP) instances to prototype their models, prior to trained models then being deployed on a NVIDIA DGX platform in order to accelerate time to results.
“This is the third consecutive year we’ve working with NVIDIA to support the European FDL event and we are already committed to be part of the infrastructure for next years’ research sprint. It is a huge privilege to be associated with such ground-breaking research in light of the challenges we all face when it comes to climate change.”
Dan Parkinson, Director of Collaboration - Scan
Related content
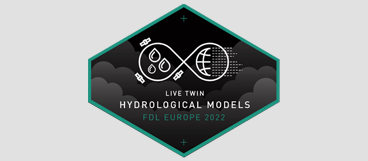
FDL Europe 2022 - Hydrological Models
Can data-driven ML models, informed by real-time satellite data build an end-to-end flood prediction system?
Read more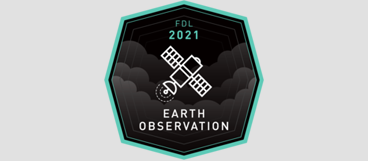
FDL Europe 2021 - World Food Embeddings
Using AI and satellite imagery to track the world’s food supply.
Read more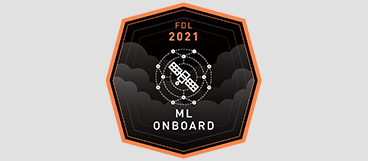
FDL Europe 2021 - ML Onboard
Using AI and satellite imagery to identify extreme weather ‘hot spots’.
Read more